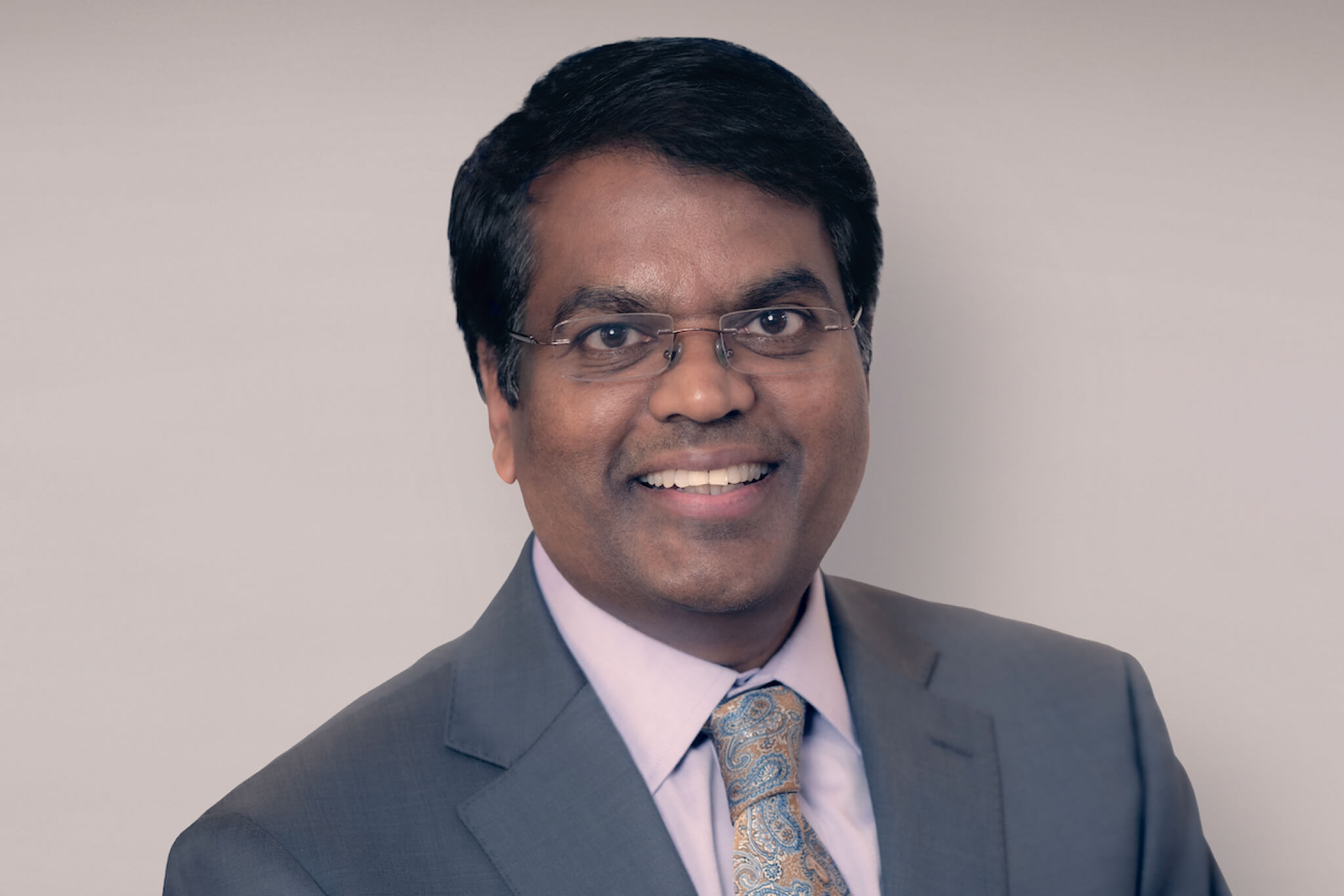
Every enterprise is becoming more sophisticated in the way it uses Generative AI.
In our series of ongoing discussions with enterprise chief technology and chief information officers, we are learning how the corporate AI journey is accelerating. In this interview, we learn about an organization where data is foundational: S&P Global. Swamy Kocherlakota, the company’s EVP and Chief Digital Solutions Officer, shared what he is seeing and reflected on what to expect next.
Asheem Chandna: What role has AI played in the operations of S&P Global? How is its impact evolving?
Swamy Kocherlakota: At S&P Global, we’ve built one of the world’s largest and most connected datasets for the financial industry. Our data and insights power decisions across the financial ecosystem, from equity and debt markets to public investments.
And without data, there’s no AI. That’s why we’re uniquely positioned to make AI a transformative force for our business.
AI helps us turn data into insights. A former CEO told me, “Insights are waiting to be discovered,” and that’s exactly what we’re enabling. AI accelerates that discovery process. No more searching for a needle in a haystack.
Now, we’re entering what we call “AI 2.0”. Early AI efforts focused on structured, tabular data. Today, Kensho, our AI and Innovation Hub, leverages advanced machine learning and natural language processing (NLP) to transform unstructured data, producing even deeper insights for our clients.
Can you give an example of how this next phase of AI delivers insights that weren’t possible before?
Absolutely. Take a 10-K document, which is hundreds of pages long, containing detailed metrics and business intelligence. Instead of manually searching through it, you can ask AI a specific question, like, “Is this company profitable?” Our advanced AI tools analyze structured and unstructured data to identify relevant sections and extract key insights. If the answer is explicit, AI finds it. AI can also suggest questions the user didn’t even think to ask.
But perhaps the most transformative shift is AI as the new user interface. Twenty years ago, working with a major vendor meant hiring expensive specialists—logical data modelers, physical data modelers—just to build an application. It was a slow, complex and costly process.
Now, AI simplifies all of that, creating a tailored interface designed around exactly what you need. If you want insights on a company’s leadership team, AI curates that view instantly. If you need to filter down to just the global management team, the process is seamless. To enable this efficiency, we’ve implemented over 1,500 robotic process automations (RPAs) across our operations, streamlining tasks like these. Traditional UIs include every edge case. AI refines this experience, allowing us to deliver products faster.
How is S&P Global using AI to address some of your customers’ biggest challenges?
If you think about the workflows most customers deal with today, they’re incredibly fragmented – analytics on one platform, reporting in another, and tracking in yet another. We’ve eliminated that disconnect. Now, we can tie the UI to end-to-end workflows that integrate our products with third-party tools as well. This is the new opportunity and new product, discovering existing workflows and stitching them together throughout our ecosystem.
How has Gen AI disrupted operations at S&P Global?
I wouldn’t call it disruptive. We believe in evolution, not revolution. At S&P Global, we approach AI as part of a continuous journey, carrying our employees along through each transformation.
We’ve built a GPT wrapper using the Azure API and we have created an internal platform called S&P Global Spark Assist, which we gave to all 40,000 employees.
What sets us apart is our strong culture of learning. We trained them on how to use S&P Global Spark Assist, and it’s now evolving into a powerful tool. Employees create prompts, which we call “sparks”, and then can share them across teams and across the organization. We have even developed a prompt library for these sparks.
We’ve also integrated this system with internal APIs, allowing low-code or no-code workflows. For example, a prompt can query an external database, pull the information back, and deliver an answer seamlessly. Employees can choose from multiple models, and entitlements are in place to ensure appropriate access.
This grassroots initiative has taken off, with employees sharing and building on sparks.
What do you see as the next big evolution in AI over the next few years?
If you’d ask me this question a year and a half ago, I would’ve said the focus was on choosing the right LLM. Six months ago, I would’ve said building domain-specific models to meet specific needs.
Today, I think the real value isn’t in the AI itself or the model, but in the applications and what you can do with them. For example, I’d like to see greater focus on process mining and engineering. If we’re using AI as the new UI, we need to understand where people are spending their time. Traditionally, methods like Lean Six Sigma were used to analyze workflows. Now, new mining tools can digitally map and optimize workflows, while also providing insights into where teams are spending their time.
The key is tying these processes together with workflow tools. Previously, we worried about how processes connected to people. Now, in a broader sense, we are linking people to machines, machines to machines, and machines back to people. Managing this workflow is the challenge and the opportunity of the future.
Can you give me an example of a machine-to-people process that doesn’t exist yet but likely will soon?
A great example is a “quote-to-cash” system.
Currently, when a customer places an order, multiple human steps are involved; verification of the order, legal reviews, contract approvals, and provisioning. Soon, AI could analyze contracts instantly and flag compliance issues with high confidence, reducing the need for human review at every step. Instead of legal teams checking every contract line by line, AI could identify only the elements that require human attention, making the process exponentially faster and more efficient.
What role do startups play in your AI strategy?
Startups play a key role in our AI project plan. We’re actively collaborating with several organizations. In some cases, we’re making investments in them; in others, we’re acquiring them outright.
Our engagement with startups evolves over time. Early on, we don’t commit fully; instead, we observe how their feature develops. As they expand into a broader platform, our level of engagement increases.
One area where startups have been particularly impactful is cybersecurity. As AI becomes more advanced, so do the threats against it. AI models can be manipulated in unintended ways—similar to jailbreaking a phone. Systems are also vulnerable to prompt injection, where bad actors trick AI into generating harmful code or executing unintended actions.
We’re using a framework from a startup that lets us manage and add protection modules over time. This is a great example of how AI startups are evolving. They often begin with a single, specific use case and then grow into a full platform.
AI technology is facing its forms of disruption and debate. How do you see recent debates about DeepSeek and AGI playing out?
I had the opportunity to attend the session at Davos where DeepSeek and the timeline for reaching AGI were discussed.
One of the most interesting perspectives came from the Anthropic CEO. He reframed the conversation: rather than fixating on the idea of AGI as machines becoming smarter than humans, think of it as having “geniuses in a data center.” The focus should be on how we use those geniuses to our advantage.
A year ago, I would have said AGI is still a distant reality. Today, I’m convinced that “geniuses in the data center” are just around the corner.
But to get there, the AI ecosystem will need to overcome three challenges:
Energy: AI needs significant energy resources to reach its potential. Governments must create policies that support the energy infrastructure needed for AI. If they don’t, they risk falling behind.
Regulation: Regulation can either accelerate or hinder AI’s development. Policymakers must strike the right balance to unlock AI’s potential while ensuring proper safeguards.
Innovation through constraints: The advancements in DeepSeek remind me of Newton’s quote about standing on the shoulders of giants. Building LLMs relies on prior innovations, curating data, optimizing architectures, and finding efficiencies. For example, they used techniques like deduplication and floating-point operations (FP8 instead of FP32) to make the model more efficient with limited resources.
What’s remarkable is how these constraints, like limited GPUs, drove innovation. They took a first-principles approach, proving that breakthrough advancements are possible even with fewer resources. By open sourcing their work, they’ve set the stage for others to build on these innovations, supporting the idea that AGI could be achievable by 2027.