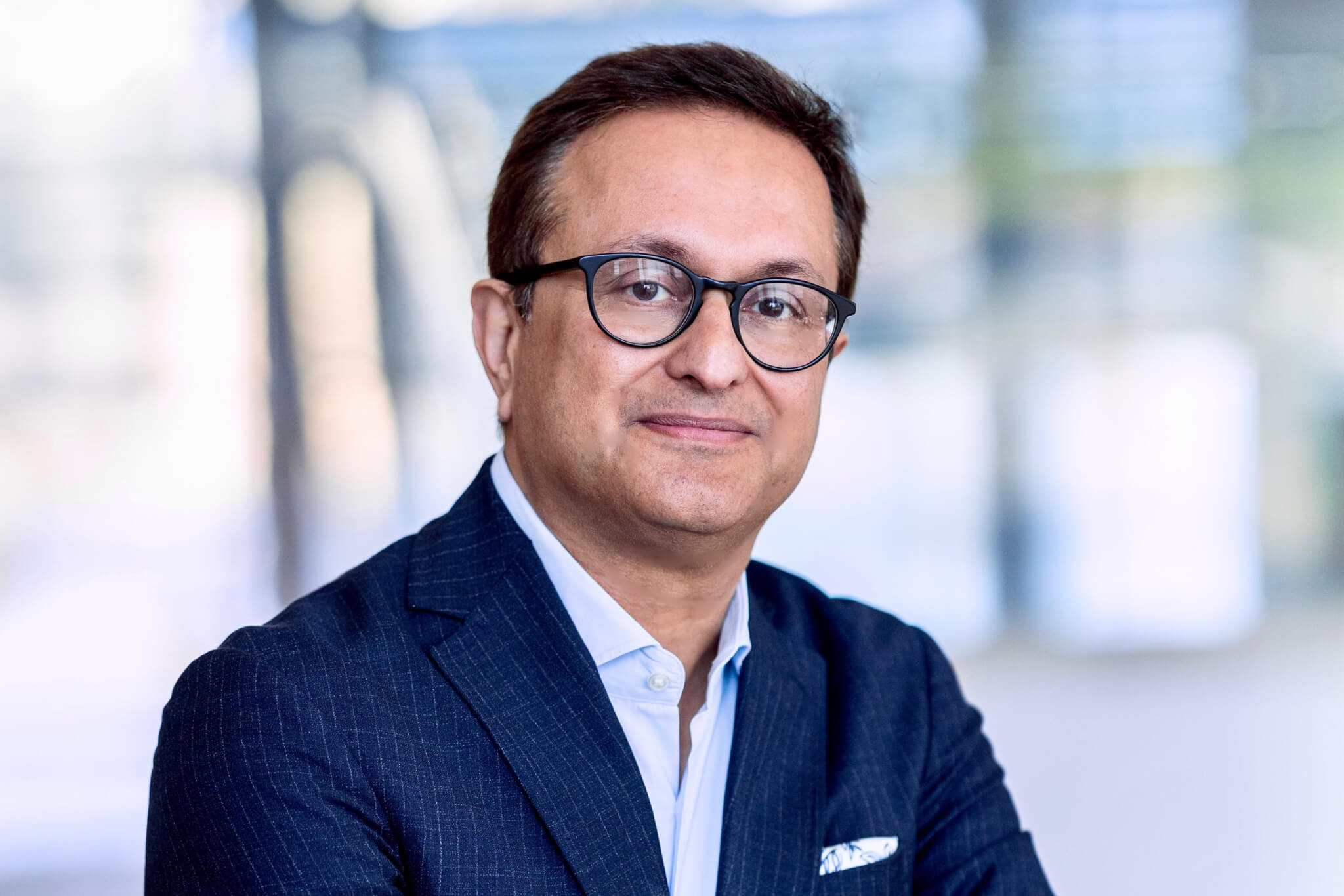
Two years after the launch of ChatGPT, Generative AI has become the most talked about and disruptive technology in business.
Adaption and early experimentation have been widespread. But to understand more clearly what role Gen AI – and AI more broadly – is playing inside large enterprises, I began a series of conversations with CIOs and CTOs at the world’s leading companies. My goal is to understand their respective AI journeys and share their views on the specific value the technology will bring to their organizations.
This first conversation features the perspectives of Bijoy Sagar, Global CIO and Digital Transformation Officer at Bayer, one of the world’s largest life science companies.
Asheem Chandna: What problem are you and your team trying to solve with AI?
Bijoy Sagar: AI is a tool that is going to transform the way we do business. But if you think of it as more than a tool, then you risk falling into the hype of looking at what it could be as just a standalone solution, delinked from the actual business problem we are trying to solve. I am a firm believer in the idea that the business case should drive where and how much AI we use.
The big potential of Gen AI is really to be able to do the things that we can’t do very well today. For example, with drug discovery — there are some serious problems we must solve, some of which we can address today, because the technology is already here with AI. Protein folding is another area where AI solutions hold early promise. Yet for other problems, the promise is still far out.
At Bayer, our mission statement is ‘Health for all, hunger for none.” AI is a great tool to help us go in that direction.
How do you see Gen AI providing solutions in the specific areas you mentioned — drug discovery and protein folding?
For early discovery, the big challenge is how to identify the right candidates as fast as possible. The sweet spot is combining other forms of AI with Gen AI. So here, we deploy AI in three different ways.
The first is foundational science, or protein folding. Twenty amino acid threads can create a trillion possible protein folding combinations. Today, we can already do pattern recognition using AI tools. Further out, with greater computing capabilities and advances in AI, we may be able to do more advanced recognition and modeling.
The second piece, which we are very much involved in today, is enhancing the ability of our scientists to do things more efficiently. Gen AI can help bring data together, so scientists can ask better, higher quality questions. They can also conduct literature searches in a more structured way. They can look at a molecular structure physically, and comb the existing research on that structure, and think through it more deeply.
Third, AI can really help us with clinical trial design and execution. A lot of companies are realizing that clinical processes are a problem. We have enough viable candidates; the main issue is that clinical processes take too long and are too inefficient. Patient selection is really challenging, so a clinical trial goes on forever, which affects the company’s ability to bring something to market faster.
How else is Gen AI being deployed in your organization?
We want to be able to bring our innovations to market as fast as possible. And that, I think, is the biggest near-term advantage of Gen AI.
Specifically, AI can help improve go-to-market strategies, maximize the ability for us to manufacture properly, help us get our offerings to the right people, and ensure patients and physicians have the right information. At Bayer, we are even using AI to train sales reps, to anticipate questions better.
On the Crop Science side, there is a lot we are doing around precision farming, crop monitoring, and pest management. We are using AI to help improve the efficiency of how we bring crops to market and address the impacts of climate change.
We see AI tools helping farmers make data-informed decisions in real-time — ensuring that data is effectively tied to planting. Ultimately, the amount of space required to produce the same yields is getting smaller.
What tangible value will Gen AI create in the next few years?
For our organization, in the next few years, we are trying to build what we call the AI-enabled enterprise. Today, Gen AI doesn’t do well with structured data. Our two-year plan is to really change this so that people can ask better questions.
With Gen AI, we now have a fairly scaled up version internally. We call it myGenAssist, and we are building essentially engineering platforms for every area – and we are connecting them all through APIs into this platform.
What trends do you foresee shaping or impacting the future of the AI space?
I have several specific predictions for the near-term future.
First, I predict LLM models are going to get commoditized even further.
Second, more of the value harvesting will happen in the customized, small language model space than at large language models.
Third, the regulatory landscape and governance of AI will change dramatically, as more countries will pass laws that restrict how we could use these tools.
Fourth, we are going to hit a wall at some point of how much data we can actually access – which in turn could limit the value we can actually access.
How is your organization approaching engagements with AI startups?
In some areas, as we have said, we are not going to use our internal systems to train, because there are startup solutions which are already tailored and effective. One example is Harvey, the startup leveraging Gen AI for help with legal. Another one is AlphaFold which helps with predictions of protein structures.
But there are yet other startups that we don’t necessarily see value in partnering with, because an organization of our size now has better in-house Gen AI infrastructure.
Interestingly, some of the startups have become value-added providers of a problem space built on AI, rather than standalone AI providers – what I like to call the Uber of AI. For example, there is one startup that helps improve clinical trials, which is built on an existing Gen AI platform. I would like to see more startups move in that direction. In other words, they’re built on Gen AI, but they’re not Gen AI companies per se.
When you look within your company, what is being disrupted by Gen AI?
First, knowledge transformation is being greatly disrupted by Gen AI. Whether it is scientists or farmers in the field – the quality of answers they get to questions asked has improved greatly.
Gen AI is also transforming enabling functions across business units. For example, procurement and finance departments complete a tremendous number of tasks which require collation of information to help drive at insights to inform decision-making.
Coding of course is another area. We surveyed all of our engineers recently – 1000s of people – and all of them found value in Gen AI coding support tools.
These benefits of Gen AI are not replacing humans. Gen AI changes the way our team members work – improving productivity and efficiency, allowing them to focus on higher quality work while automated systems help with the rest.
Is there something you wish could be solved by Gen AI or AI that has not yet happened?
The biggest unsolved issue is tackling internal data in large companies — how do you bring that data to a point where it can really be used for training?
Another opportunity is the automation of ethical use of AI monitoring and compliance.There is no company today that can plug and play in the space of ethical use of AI — automated monitoring of models, flagging of challenging issues, etc. I hope to see an engine that could be run on all of our models and no human being has to worry about it. The AI engine would validate the use of AI for human beings or inform us when we must comply with restrictions.