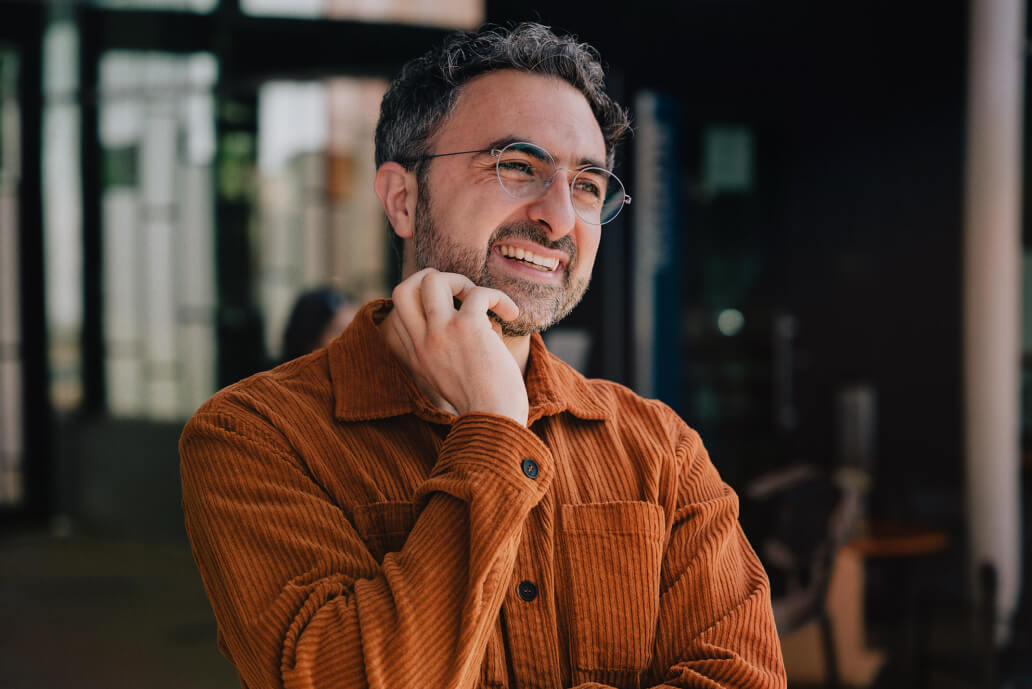
We are thrilled to share the news that Mustafa Suleyman is joining Greylock as a Venture Partner. There are few people who are as visionary, knowledgeable, and connected across the vast artificial intelligence landscape as Mustafa. At Greylock, Mustafa will spend his time advising early-stage companies and investing in promising startups in the AI space.
Mustafa joins us from Google, where he was Vice President of AI Product Management and AI Policy. Before that he co-founded DeepMind, the world’s leading artificial intelligence company, which was acquired by Google in 2014 for $650M.
I’ve known Mustafa personally for more than a decade. We first met at a pub in London. While I have some fun memories of that night (including trying my first “toad in the hole”), what struck me most was our inspiring and provocative discussion on the power and potential of AI to help solve some of humanity’s most urgent challenges. It marked the beginning of a deep and lasting friendship between Mustafa and I.
There’s no doubt that AI is one of the most transformative technologies of our time. Mustafa has been at the forefront of some of the most exciting advances in this space. During his time at DeepMind, Mustafa led teams inventing and deploying cutting edge AI systems to more accurately detect breast cancer in mammograms, to diagnose 50 different eye diseases in OCT scans, and to control Google’s multi-billion dollar data centers to optimize energy consumption. He also worked with many teams across Google to apply the latest AI techniques in Android, Hardware, Play, and Cloud.
Over the years DeepMind achieved many groundbreaking contributions to the field of AI research and applications. Most notably DeepMind developed AlphaGo, an AI system that beat the world’s strongest Go player in a now legendary multi-day competition broadcast live and watched by millions of people across the world.
Moreover, Mustafa and I share a common love of philosophy, which drives how both of us approach our work in technology and entrepreneurship. At its core, philosophy focuses on improving our understanding of humanity, and how we evolve as individuals in a society. Mustafa has spent years thinking about how technological advances impact society, and he cares deeply about the ethics and governance supporting new AI systems.
What’s more, I know Mustafa is a builder at heart and he is excited to spend time with founders and to explore new ways that AI technology can make an enduring difference in the world.
Recently, Mustafa joined me on Greylock’s Greymatter podcast to talk about the current state and future of AI, his reflections on building DeepMind and his time at Google, and what’s next for him. You can listen to the podcast here.
Episode Transcript
Reid Hoffman:
Hi, and welcome to Greymatter, the podcast from Greylock, where we share stories from company builders and business leaders.
I’m Reid Hoffman, partner at Greylock, and I am thrilled to welcome the newest member of the Greylock family, Mustafa Suleyman, to the pod.
Mustafa Suleyman:
Hey Reid. Thanks for welcoming me to the podcast. It’s great to be here and I’m also very excited to start at Greylock.
RH:
Mustafa and I have known each other for over 10 years. For those of you who need a quick summary on Mustafa, he is a world renowned expert in artificial intelligence. He’s one of the co-founders of DeepMind, an artificial intelligence lab in London that was acquired by Google in 2014, for $650 million. And in the last couple years, he’s been a VP, working on AI Google.
Today we’re going to spend some time across a whole wide variety of topics: philosophy, current state and future state of artificial intelligence, reflections on DeepMind, being an entrepreneur, your time at Google, and what’s next to you as an entrepreneur.
But part of the reason I’ve been looking forward to this for years is that I know you personally, and as a friend, and what I have learned so much in so many different ways is the kind of questions around society and humanity and technology and governance and putting all these things together.
I’ve been looking forward to this direct journey of working on artificial intelligence together because we’ve been working on it in so many other contexts.
And while normally one might start with the bio and DeepMind – we’ll get there because it’s really interesting – I think one of the things that we should start with, because we have a shared interest in it, is philosophy and how philosophy leads to kind of shaping technology and shaping technology for humanity. And it’s one of the things that I think has perhaps been kind of under-discussed with you.
So let’s start. How did philosophy launch you on your path towards technology?
MS:
Yeah, that’s a great place to start. So back when I was doing my undergraduate degree in philosophy at Oxford, the big thing that I loved about that training was that it helped me to become a systems thinker – to think about, structurally, how the big chunks of our world at every layer of abstraction, from our inner experience that then leads to creating our social relations, which then leads to the way that we create ideas and culture, how this flow of information and ideas then goes back and gives us top-down causality and actually shapes who we are as people.
And that cycle of feedback and interconnect actually has some very interesting parallels to the way that we design software and technology platforms at scale today.
In many ways these platforms represent a set of values that product designers and engineers have. And when they set out to create these things, they go and deliver a product or a service that is hopefully useful, it’s fun and entertaining or informative. But in doing so, it shapes behavior. And I think that that’s a helpful way to think about how we can try to create technology, which really serves us well and collectively helps to move humanity forward in a positive way.
RH:
So what was the aha moment that said, “Actually in fact, technology is a path to greater humanism and possibly ways of really helping society”?
MS:
Yeah, it’s a great question. I mean, as much as I was interested in the structural side of philosophy, understanding the nature of the human and our social relations, I was also very much interested in moral philosophy. And as a committed, effective altruist, even at the time, I was always thinking, “How do I use the time that I have on earth to have the maximum positive beneficial impact?”
And that came with driving my motivation to drop out and start a charity, which I ran for a bunch of years. And I then went from there to work in local government, hoping that I could sort of scale up the influence and effectiveness that I was having with nonprofits.
And over time, I then quickly realized that, actually, the real thing that I wanted to do was around conflict resolution and figuring out how we can run these large-scale, multi-stakeholder change labs, which I was doing in 2005, 2006.
That led me to the climate negotiations in Copenhagen in 2009. We were convening a huge group of academics and researchers and nonprofits who were involved in one of the nine negotiating tracks, [in this case], reducing emissions from deforestation. And we were trying to align all these different people to get them to have a consistent negotiating position with the states.
And as I’m sure many people will remember, it was actually the first year that Obama was going to make a very big speech and hopefully make a big commitment. And unfortunately it was all very disappointing and no agreement was reached.
And I think in that moment, I basically realized how difficult it is for us to achieve consensus and deliver these large-scale agreements in the world in order to make progress on our tough social problems.
And the funny thing is that in parallel, I was sort of watching the rise of Facebook at the time. I think it was only a few years old and maybe in 2009, I think it had like a hundred million monthly active users.
And I was just totally blown away that a new technology company, a new platform that was maybe only three, four years old at that time, could have brought together a hundred million monthly active user and was shaping the way that we think and sort of influencing the way that we connect with one another and so on. And that was just profoundly inspiring to me.
And that was when I sort of realized that technology was really the most important thing that was going to happen in my lifetime. And I wanted to be right at the center of that. And so that’s how I sort of set off looking for some co-collaborators and co-founders for a new technology endeavor.
RH:
So say a little bit about that. Because one of the things that I find really amazing and fun about your journey is that you turned to an area – artificial intelligence – that was deep and prescient. And how did you go about your process of going, “Okay, technology is a way of saying the future can be shaped importantly for humanity?”
MS:
Yeah, I mean, like most people, I guess, I set about on a quest to find like-minded [people] who could teach me things and who I wanted to collaborate with.
And that led me to Demis Hassabis, my co-founder at DeepMind, and he introduced me to our third co-founder Shane Legg. They were both working on their PhDs and post-doctoral work at the Gatsby Computational Neuroscience Unit at UCL in London at the time. And they kindly invited me to come to some of their lunchtime seminars. And I ended up just spending a bunch of time with researchers who were working on what was called machine learning.
At the time, it was kind of taboo to say that you were working on AI, which seemed super far out and wacky. And we had just come through the sort of AI funding winter, where it was really difficult to get research funding for AI, but nevertheless, Shane to his credit, had spent his entire PhD working on a definition for intelligence. And he had looked at 65 different definitions from a wide range of different cultures and sectors for what it is that actually makes up intelligence. And he had aggregated these into a single formulation and turned it into an engineering problem.
And this was the kind of key thing that probably [led to] my first moment of optimism that this might be a tractable problem to work on. Shane, for his PhD, had articulated a way that we could actually (in a very, very, sort of engineering-focused approach) measure the progress that we were making towards systems that were more intelligent. And that felt very, very promising. Even though it was extremely nascent, it felt like a great place to start.
RH:
And, so this is 2010, if I recall.
MS:
Right, exactly. Yeah. 2010 in the summer. And very much at a time when most people were trying to work on very narrow applied problems for machine learning. And Shane was very much focused on, “How do we take the big theoretical question of defining intelligence and then operationalizing it?”
RH:
There had been a bunch of very good academic work, but the effort to actually make an effort to go very deep and compute and to be broad was not yet kind of the common technologist wisdom, which it is now.
It’s one of the most amazing technology efforts in, I think, the world, and definitely in Europe and London. When did you get your aha moment that, “We are going to build something new that the world hasn’t seen” ?
MS:
Well, I remember one of the first moments that really got me excited was when I saw us make progress with learning to identify numbers – handwritten digits from an image. And that sounds like a really simplistic problem, but back in 2010 and 2011, most of machine learning was characterized by what’s called handcrafted feature engineering. And so engineers would literally sit down and define the optimal shape and angle of lines and edges in order to be able to identify objects within images. And that handcrafting process is very brittle and it doesn’t scale well, and doesn’t generalize to new environments that your AI hasn’t seen before.
So this new wave of approaches was trying to train an AI system to learn its own representation of good edges and lines to better detect objects scenes. And in a very, very simplistic way, the team was trying to do this for digits.
And what I saw was a short video showing the learning process for how it was doing this classification. So it would go from a very blurred mushy, black and white representation to resolve quite a distinct, say a number seven, for example. And it looked pretty sharp. And I was like, “Wow, that’s really encouraging. That’s the first time an algorithm has learned its own representation of digits.”
And over time, a few years later, the team combined this with reinforcement learning to play the classic Atari games to superhuman level performance. And that in itself was like another incredible moment for me. It was pretty remarkable. I mean, I remember standing in the office watching the learning process for our DQN algorithm, our deep reinforcement learning algorithm play the game of Breakout, and many listeners will know this game, but you basically get a control, a paddle at the bottom of the screen, and there’s a ball that bounces up to knock the bricks out. And the more bricks that you knock down, the more score you get. And in this case, the DQN was given just the score and the raw pixels to try to learn a relationship between pixels and the control actions of moving the paddle left and right. And the amazing thing was that it had discovered this incredible strategy of really efficiently tunneling a route up to the back so that it could get behind the bricks and get the maximum score with kind of minimum effort.
And this was the first time I saw an example of a system that could learn its own representation of what was valuable and rewarding, and in many ways learn knowledge that wasn’t available to many other humans. Like many regular players, they didn’t discover the strategy. I certainly didn’t discover the strategy. And that was the holy grail to me. I was like, “Okay, we’re really onto something. This is an example of something that can learn new knowledge.”
And that’s obviously the real attraction of building these AI systems; that they could potentially learn new insights that could help us do great things in the world.
RH:
Yep. And obviously this kind of thing is counter to the classical stereotypes that machines can’t learn creativity and can’t learn new things; that they can teach us. And I think that naturally leads to the kind of epic moment by which I think DeepMind blazed [out] on the stage, which is the AlphaGo Lee Se-Dol moment. So please share that with us.
MS:
So, Go is played on a 19 by 19 board with black and white stones. And the objective is to try to surround your opponents stones with yours. And then you take them off the board and the rules are really as simple as that. There’s nothing else to it. But the complexity of the game is phenomenal. I mean, there are 10 to the power of 170 possible configurations of the board, possible state spaces. So the traditional methods of search through all the different options just don’t work because you don’t have the compute [power] for that.
So the algorithm really has to learn clever strategies to navigate that search space. And the way that we trained AlphaGo was that we first gave it 150,000 or so games from human experts. And we said, “Okay, learn from the corpus of the best possible experts we have.” And it was great. And then played reasonably well after that point. But the key insight was that we then basically spawned a whole series of instances of AlphaGo and we got it to play against itself.
In doing so, it was able to simulate millions and millions of new games, which obviously had never been played before, and therefore efficiently explore the space of all possible games. And then of course, we’ve set it loose by playing Lee Se-Dol the world champion of Go at the time in Korea, in this incredible live match over the course of five days and ultimately AlphaGo won.
The amazing thing there was that it learned some incredible new strategies that had never been discovered before. And that was the holy grail for me. I was like, “Okay, we really can train an algorithm to discover new knowledge, new insights. How could we apply that? How can we use that training method for real world problems?”
RH:
Yep. I completely agree. And this is, I think, one of the places where DeepMind has been one of the strong contributors to now what I think is a broad renaissance in the next generation of evolution.
One of the things that I really appreciated about your focus was How can it be applied? How can these applications of artificial intelligence be something new and important in the world? And that was one of the functions that you did as a co-founder of DeepMind. Could you go into the applied areas sense?
MS:
“That was my motivation all along; to try and see how we could use these systems to do things in the world and really make the world a better place.”
And so as part of that, we took aspects of the methods that we were developing, particularly around applied deep learning, and using them to do things like identify cancer in mammograms, identify, in one case, 50 different blinding eye diseases from OCT scans. We used it for head and neck cancer, radiotherapy treatment.
And we also did applications in climate change as well, where we tried to use these sorts of methods to manage the Google data center infrastructure more efficiently, and we reduced the amount of energy required to call the Google data center infrastructure by 30%.
And so, it was very exciting that we could be able to apply methods that had been developed in gaming environments,to transfer them over to the real world. And I think now the field is on fire in terms of the number of startups that are applying deep learning methods to all kinds of awesome problems.
RH:
Yeah. I completely agree. And I think one way of looking at it is that most folks kind of understand that software is already transforming the world, but AI and cognitive capabilities are very broadly creating an exponential level of impact. because they’re – by depth of compute, by self play, by data – doing enormous generations of of capabilities of artificial intelligence capabilities that just weren’t there before.
So what are some of the areas that you would think have been most transformative, and give us a sense of what is launching us into the future?
MS:
During the time that we made a lot of progress at DeepMind working on games and simulated environments, I think the key breakthrough that we made was demonstrating that self play in simulated environments, where there was a clear reward signal could produce incredibly powerful systems. And that has definitely been a source of incredible research over the years.
But I think the big breakthrough in the field, more generally, that took my breath away was when OpenAI scaled up the Transformers breakthrough that Google had developed in 2017.
So there was a paper developed at Google which was essentially a way of doing more accurate time series prediction and being able to generate from that sequence. A couple years later, what OpenAI demonstrated was that they could actually scale up this work using very large-scale compute, and really produce natural language generations that looked incredibly human-like. That really was, I think, a great step forward in the field, and I think it is a sign of what’s to come.
RH:
On the Transformers, go a little bit into more depth. What is the way this takes a large corpus of data, trains into something interesting, and then what could be really interesting in doing, as you begin to look across back to applications that change humanity?
MS:
So if you think about it, most information in the world has a sequential structure. Each piece of data is in some way related to data that has come in the past, and a big part of what makes us intelligent is that we can learn, as humans, abstract representations of a series of things that have happened in the past well enough that we can reason over these elements. In some sense, this is what Transformers do. They take sequential data, build a good model of that data to learn an abstract representation of it, and then they use that to try and predict what’s going to come next.
So for example, in the case of language generation, what we’d really like to do is give it a sentence to predict which word is likely to come next. So that was one of the big areas that these have been applied to.
The trick that was discovered was this thing called attention. Now, when you parse a sentence, when an algorithm ingests a sentence, it has to try to assign a weight to each one of the individual words that describes something about the importance of that word with respect to adjacent words in a sentence. And that mechanism to learn attention – to attend to the salient features in a sentence in order to produce a good representation of what that sentence is – is actually the big breakthrough that came with Transformers.
Language is very, very confusing. So if you take… For example, “The 44th president of the United States was Barack Obama,” the algorithm has to learn that Barack Obama is both related to the United States, is the president, and related to 44th, and so each one of those has some weight. That’s basically the big challenge of natural language understanding and natural language generation.
I think what was demonstrated by the scale-up of GPT-3 is that we can now start to do this well enough that you can generate very plausible, full sentences, in fact, entire paragraphs. That’s very exciting, because I think it’s the beginning of machines being able to communicate with humans in our language, rather than us having to learn the language of computers, if you know what I mean.
RH:
Yep, exactly.
So language is obviously part of what GPT-3 has done. It’s also, of course, done Codex, and Copilot. What are some of the areas when you begin to go, as it were, multimodal, right? You say, “Okay, we have these Transformers and language models. But actually, in fact, it’s going to apply a lot broader than you might think.” What are some of the gestures and lenses that you can give people who are listening to see how much AI is a transformer of the world?
MS:
Yeah. So some people have actually started to refer to Transformer models as foundation models, precisely because they have this multimodal character, which is that the same core architecture – that is, one that encodes a representation of the input data and then decodes a representation to generate a plausible output. The same core architecture can be used for a whole range of different modalities.
I mean, so you take images, for example. We’re getting really good at generating images that have never been seen before, given some large corpus of training data. The really cool thing is that you can jointly embed the language, the audio, and the images in the same representation, such that you could write in natural language, say, “Generate me an image of a blue car in the shape of a crocodile.”
There is some representation that can be generated that is some joint space in between those three different ideas: the color, the car, and the crocodile. You can also go the reverse. You can ask it to transcribe a description of what’s in the image. That’s very, very exciting because it starts to look and feel quite human-like in the way that we store and represent ideas. We think of colors and shapes and objects and language as associated. So that starts to feel like a pretty plausible way that you can build very, very capable systems.
That’s why I think people are starting to call these things foundation models. But of course they require a huge amount of data to train them, and a lot of expertise and so on, and a lot of compute. I think they’re definitely starting to come of age.
RH:
If you’re a founder, a technologist, and inventor, you’re looking forward to two or three years about how these Transformers and how these foundational models are going to be creating new kinds of capabilities for machines that hasn’t been seen before. Cognitive functions that are original, and creative, what’s some of the “shining a light forward” stuff that you’d say, “Hey, entrepreneurs think about X, Y, and Z”?
MS:
“There’s no question that at least in five years time, these technologies are going to be completely ubiquitous.”
In fact, if you think about it from a founder’s perspective, these are like a new clay. They’re the new tools that are going to allow us to create all kinds of new experiences. The fact that we will be able to generate perfect audio, perfect images, perfect text, even video, and be able to control the way that is generated rather than hand-scripted, right? You should be able to give an instruction in natural language for the generation of this new content. I guess, your imagination is the limit of what can be produced.
I think if you just look, for example, at the rate of development in the amount of compute that is used to train these things, it’s pretty incredible how that has been increasing exponentially. In the last 10 years, the largest AI training runs have been growing exponentially with a three month doubling time.
So just to put that in context, everyone’s familiar with Moore’s Law. The doubling time for Moore’s Law is actually a two-year period. So this metric has actually grown by more than 300,000 times in that period. Whereas if it was actually Moore’s Law, it would’ve been like 7X.
So that gives you a sense of the rocket ship of a trajectory that we’re actually on. The good news is that whilst we’re taking huge leaps forward in the amount of compute used, the algorithms themselves are also getting dramatically more efficient. That means they will be cheaper to run and more ubiquitously available. They’ll be able to run on device. You’ll be able to spin up your own instance of them. You won’t just have to rely on a cloud service.
That’s, I think, probably the most exciting thing. They’re getting easier to implement. Everyone from non-technical social media influencers and content creators all the way through to enterprise software builders will be able to use these tools to create new services and products.
RH:
Let’s shoot back a little bit to the co-founding and the path of DeepMind.
One of the things that I thought you and Demis and Shane just did spectacularly well was to build this amazing technology company in London – in Europe, not in the Valley. There’s all these network effects that really help Silicon Valley companies. So what were some of the key lessons about building out DeepMind from the early days and doing so in a location that’s remote from the network effects that we get from being Silicon Valley entrepreneurs, and investors, and technologists, and so forth?
MS:
Yeah. I think we had a pretty unique mission If anything, being in London helped us to be a little bit more distant from the pace and energy of the Valley, be maybe a little bit sort of slower and so on, I think the kind of people that we were looking to attract were researchers who might have been a little bit more cautious about joining a typical startup.
I think our emphasis was to try and create the perfect research environment where the very best researchers in the world were sort of surrounded by all of the engineering support and expertise they needed to maximize the ideation process, then surround that with a layer of applied research engineers and some applied product people who could then take those breakthroughs to market (albeit via Google, and that was our only customer).
Google was actually brilliant for that, because Google was like a microcosm of the rest of the world. It’s such a huge place, and there are so many different types of product application areas, all the way from wind farms that we worked on, right the way through to content recommendations and so on. That was just a really neat frame for us, because we could have the benefit of being small and outside of the buzz of it, but also very much focused on our core mission at the same time.
RH:
Well, one of the things towards the end of the DeepMind journey, after being merged with Google, you had obviously encountered some controversy. Some news came up about an aggressive management style. I remember talking to you about this, and I feel that I think it’s really important to share this part of your journey too, because I really love working with people who are infinite learners, and people always assume that infinite learner is just like technical skills and management skills, and isn’t the full spectrum. So say a little bit about what circumstances you found yourself in and then how you adjusted.
MS:
Yeah. I’m glad you brought that up. I mean, I had a period in sort of 2017, 2018, where a couple of colleagues made a complaint about my management style, and I really screwed up. I was very demanding, and pretty relentless. I think that at times that created an environment where I basically had pretty unreasonable expectations of what people were to be delivering and when, and ended up being pretty hard charging, and that created a very rough environment for some people. I remain very sorry about the impact that that caused people and the hurt that people felt there.
I think from my side, it gave me the opportunity to really take a step back and reflect and grow and mature a little bit as a manager and a leader.
I think I’ve always been very focused on the big picture and sometimes not very attentive to/or sympathetic to the details, and super-focused on speed and pace over being caring and attentive to how people are feeling in the fast-paced environment.
Yeah, I’ve been working with a coach for the last few years and that’s been a fantastic experience. In fact, just recently, my coach interviewed a bunch of my reports from DeepMind and a few other people and got a whole bunch of their feedback, and it’s given me a lot of very constructive things that I’ve been working on and trying to improve. I realized it’s just going to be a lifelong process of learning and improvement, and so I’m making that a part of my practice every week of learning and reflecting with my coach and with others.
It’s just great to have somebody that can help me to constantly learn and grow and constantly get feedback from people. And I think it just sets me up to be constantly in that mode of reflection and learning and improvement, rather than seeing it as a finite one time effort.
RH:
Yeah. I completely agree, and part of the reason I wanted to linger here a little bit is not just so that people can see you for the committed human being that you are, but also that this is a good thing for everyone to learn from as part of thinking entirely about infinite learners.
So, let’s come back to another part, something I was learning from you, from the early days when we were getting to know each other, which is this focus on what is frequently the alignment problem in AI, or AI safety. Or, how do we get good governance around this critical technology, that it’s not just a one technological entity that might be trying to drive a whole lot of profits, but how do we both make sure not only are the benefits well distributed for society, but also that the participation and the governance is good?
MS:
I think the first thing to say about this is that it’s very much a work in progress. I mean the field is really just trying to figure out how to even begin to think about governance in this space. And I think what I feel good about is that we’ve spawned a flotilla of different experimental approaches.
So we tried to think about the legal structure of Deep Mind and how that might be set up in a way that inherently introduces more oversight and accountability into our development process when we’re working AGI. We’ve experimented with different oversight boards, with different ethical charters, with types of research. And a bunch of other companies have tried to do that as well, and there’s been a whole sweep of academic research departments that have been started non-profit institutes over the years.
I think it’s all been good, but I definitely feel that we haven’t really come close to cracking this nut of how we make technology platforms, software, and of course AI feel like it’s happening with people, and where people have significant influence in shaping how it arrives in their world and doesn’t just happen to people.
“This is going to be the big governance and ethical question of our age in the next couple of decades, because the technology is obviously developing really rapidly and things are changing so fast that I think figuring this one out is going to be the big one for humanity.”
RH:
Yeah, I think you’re totally right. It’s kind of you don’t wait for perfection, you have to take multiple shots on goal. You have to try things. Part of how deeply built-in to how you guys were thinking about this is that when you were bought by Google, you said, “Look, part of the deal has to be creating an internal AI ethics board. It’s really important.”
So, whereas most people think all you’re trying to do is maximize comp or acquisition price, or roles and responsibilities – No, no, no, actually this has to be there as well. Say a little bit about the thinking, and obviously it must have been a little nerve-wracking since these other things matter too, in terms of how the organization will be working. What were some of the lessons of that process, and what pointers would you give to other entrepreneurs who are taking an ethics- foundational approach?
MS:
I mean I tell you what, if Google thought we were pretty nuts to be working on AGI in 2014, they certainly thought we were nuts that we wanted an ethics and safety board to help us manage the long-term consequences of super intelligence. And so it was definitely pretty wacky.
Luckily, I think in Google we found a partner that also believed that this was the future. And I think looking back on it, I feel very humbled by it. We made a lot of mistakes in the way that we attempted to set up the board, and I’m not sure that we can say it was definitely successful, but I do believe that radical experimentation is essential here. I think everybody should be trying to think about how they answer this question, and as soon as they’ve made some progress, or as soon as they’ve screwed it up, we have to share with the community as quickly as possible. Because what we do need is new forms of governance and new forms of oversight that are fit for the modern age.
RH:
So, after a number of years working at DeepMind and then more broadly working at Google on large-scale models and contributing across the entire industry and society on safety, alignment, regulation and governance, you’re now shifting over from being an operator to also being part of the venture firm and investing. I know you’ve been doing some investing in the past, so talk a little bit about your experience in investing so far, and then bridge that to what kinds of things you’ll be looking to do at Greylock.
MS:
I think the thing that I really love about doing the investing that I’ve done is that I get the opportunity to spend time with people who are visionary and fearless, and that really energizes me. I’m definitely somebody who likes to take risks and try to learn from my mistakes and try to be fearless about that. And I find it super energizing when I’m around people who also have a courageous vision of the future which sounds wacky or implausible, but are prepared to dedicate their lives to giving it a shot. And they’re the kinds of people that I like to back.
And I think that’s what we need. We need more people who are prepared to try and do bold things and tackle hard problems to try and improve our world. So I think it just makes total sense for me to align my investing with Greylock, you guys obviously have an incredible portfolio of people who have been visionary and done just that. So, spending more time with those portfolio companies, hopefully being able to extend my network and introduce it to what you guys already have will also be great. And also looking forward to working with some of your awesome partners like Saam and Sarah too, so I can’t wait to be part of the gang.
RH:
And we all could not be more excited.
And obviously as this discussion has shown, artificial intelligence is one of the massive new platform changes, maybe the most important technological transformation that affects industries, society, work, in my lifetime, in our lifetime.
Mustafa, as always awesome to talk with you. Thanks for sharing the insights. It’s so much fun, I look forward to doing this multiple times and I am so glad we get to work together much more closely now that you’re part of Greylock.
MS:
Thanks so much, Reid, I’m super excited to be joining the firm and I think it’s going to be a huge amount of fun. I can’t wait to get going.
RH:
That concludes this episode of Greymatter. To find all of our content, checkout our blog at greylock.com, and follow us on Twitter @greylockVC. You can read a transcript of this episode on our website, greylock.com/blog. And you can find all Greymatter episodes by subscribing on SoundCloud, Spotify, or wherever you get your podcasts. I’m Reid Hoffman, thank you for listening.