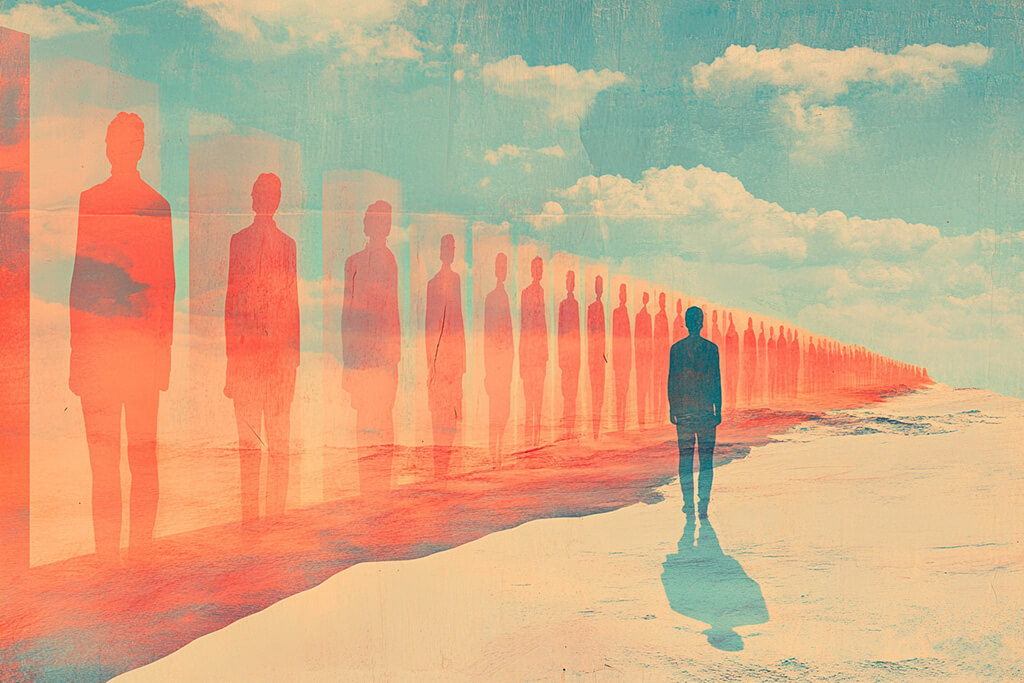
As a follow-up to my post last year on securing GenAI, I’ve been exploring the counterpart – how is GenAI changing the offensive landscape and how should organizations respond?
Deepfakes constitute one such offensive challenge. Deepfakes grabbed social media headlines again recently given the images generated by Grok’s low-guardrail image generator and the risk they present to election globally, but they also present a major issue for enterprises.
Deepfake attacks represent an advanced method of social engineering that, as of now, has been infrequently encountered in real-world scenarios. However, even for those with a solid security framework, deepfake technology is improving rapidly and becoming increasingly cost-effective and accessible. It is essential for enterprises to reassess your threat model to account for the potential risks posed by deepfakes.
I spoke to CISOs and heads of fraud at some of the country’s largest financial institutions and media companies to understand the risk presented by voice, image, and video deepfakes, the solutions being developed in the market, and the factors that founders building products should take into consideration.
Fraud and customer authentication
Unsurprisingly, deepfake fraud is top of mind for financial institutions. Voice is a commonly used authentication paradigm by banks – many of us have experienced calling into our bank and verifying our identity by repeating back, ‘at ___, my voice is my password.’ Many private bankers also rely on recognizing their customers’ voices outside of the authentication stream. Images like selfies are commonly used for verification as well and matched to IDs in financial transactions.
So far, fraud leaders at large banks report relatively low incidence of deepfakes. The approach to fraud is different than in security – security is often about avoiding black swan events, while fraud is about navigating death by a thousand paper cuts on small transactions. Accordingly, so long as losses due to deepfake fraud stay in a tolerable range, banks can take a wait-and-see approach on implementing solutions. In particular, there is concern about false positives or introducing friction to the consumer experience, as customer dissatisfaction can have a more significant impact than small fraud.
However, as voice and image prove unreliable (OpenAI recommends removing voice altogether as a paradigm), there is strong consensus among financial fraud leaders that deepfake attacks are likely to ramp up all at once – as well as a need to be ready for that time. Further, several believe there is a generational opportunity in solving this authentication challenge for humans akin to PKI on the web 25 years ago.
Social engineering
Voice and video can be combined with more traditional targeted social engineering attacks to gain malicious access to enterprise environments, conduct wire fraud, etc. Many protocols for access requests or wires include calling or video chatting the party for independent verification. With voice cloning and even increasingly realistic avatars, a handful of more sophisticated attacks have been perpetuated.
Take the cybersecurity firm KnowBe4, which unknowingly hired a North Korean spy who used AI and a stolen identity to get through the interviews and background checks. The firm detected the breach when the new employee immediately installed malware on their corporate device. When questioned about this behavior, the individual vanished. Upon investigation, it was revealed that the laptop had been mailed to a U.S.-based laptop farm, and the malware enabled remote control back to North Korea.
There’s also the example at Ferrari, where an executive received a deepfake phone call claiming to be the CEO. The target sensed something was amiss and asked the AI avatar a question only the real CEO would know the answer to. The call ended promptly and Ferrari spun up an investigation. And there’s the Hong Kong example where the attacker apparently succeeded in impersonating an executive.
These attacks are also likely to be harder to detect than many of the lower-value fraud ones. While voice cloning from a website like Eleven Labs has a distinctive and detectable signature, attacks from bespoke technology developed by hacker labs will not. While these attacks may have gaps now, given the rate of improvement of AI technology they may become more and more dangerous.
Brand devaluation
Beyond the societal damage done by fake content and news, companies run the risk of consumers losing faith in their brand. Fake videos impersonating CEOs and executives have already been put out proliferating scams or encouraging people to buy certain securities. While the financial damage may fall to the consumer, companies and public individuals wish to avoid the negative association with fake content.
On the media side, companies similarly worry about loss of faith in their brand and reporting. If consumers can no longer associate certain reporters and brands with factual reporting, media outlets risk losing their audience.
We’ve seen a number of approaches to solving the deepfake challenge.
Detection
A number of startups are working on detecting AI-generated content as well as edited images. Reality Defender won most innovative startup at this year’s RSA conference and offers an ensemble of models to test for signs that an image, video, or voice has been generated or manipulated. Some are visible to the human eye (e.g., are eye movements inconsistent/unnatural), while others are not (e.g., looking for inconsistencies in the image frequency domain).
Reality Defender and other companies like Clarity AI and Get Real Labs offer these tests via API or web interface, and they can be run in real-time or as an investigation. One T1 bank plugs a detection suite into their VOIP to monitor calls between their private wealth management team and its clientele to monitor for signs of manipulation. Media companies use the tests as an approach to conduct investigations if images may have been generated or doctored. This domain is known as semantic forensics and has drawn interest at the government level as well as DARPA’s SemaFor program. Semantic forensics combines detection algorithms with attribution algorithms seeking to infer if a piece of content originated from a particular organization or individual and characterization algorithms which aim to identify any potentially malicious intent.
Provenance and watermarking
On the big tech side, solutions target provenance and watermarking at the point of creation. C2PA is a consortium backed by Adobe, Google, Microsoft, OpenAI, the BBC, and others focused on provenance. A camera with C2PA running on it encodes metadata like the photo’s location, photographer, date, etc., in a manifest bound to the content. Any edits made in C2PA-software are recorded in the manifest as well; if other edits are made, the manifest won’t match when inspected. OpenAI is embedding C2PA in Dalle-3 and SORA.
Watermarking follows a similar goal, embedding a stamp into the content itself. Deepmind uses SynthID in their generative tools. For text, the probability of next word generation is adjusted slightly such that overall quality is not affected but for sufficiently long text it can be traced back to Google. Waveforms imperceptible to the human ear can be added to audio, and pixels or frames for images and videos. These techniques can be robust to common editing and show that not only was the media generated, but from where.
Of course, the lack of provenance does not necessarily prove something is fake or generated, only that something may be real; similarly, watermarking can be used to detect deepfakes generated from the most popular tools, but again, the long-tail or more bespoke tools may not implement it. They provide an opportunity for users to validate something, but do not prevent many cases of fraud. Provenance does help with a related issue that is actually very common, cheapfakes. Cheapfakes are images/videos that are real but relate to a different context, time, or place than implied or stated. Provenance and watermarking provide the metadata to validate the correct context.
Hygiene and education
New technical solutions should be complemented with existing technology and process hardening processes. Many banks indicated that they plan to double down on user behavioral analysis as statistical matching can capture signs of fraud outside of the deepfaked media. And correct process controls should be cemented, including device-based 2FA, predetermined questions and answers, and certain activities shifting in-person.
Teaching effective deepfake awareness represents a significant opportunity for the industry. Like the established practice of training teams to recognize phishing emails through targeted training and ongoing simulations, there is an opportunity to develop programs focused on recognizing the telltale signs of these social engineering attacks.
What does this mean for startups building in the space?
Founders building solutions need to navigate several challenges:
- Staying ahead of the cat-and-mouse game that is the constantly-improving AI technology. Taking a page from cybersecurity here makes sense in designing security and vulnerability research teams dedicated to devising and incorporating the latest methods, as does a threat intel approach to understand the different groups and attack signatures out there.
- Determining whether products should add workflow or remain only as a core detection engine. While there may be sufficiently differentiated IP in a core detection API, companies should consider workflow in order to avoid potential margin compression against other detection solutions with reasonable performance. For example, considering more holistic social engineering and impersonation attacks across modalities, or using deepfake detection as a wedge into creating a broader modern fraud solution.
- Being thoughtful about market timing and design partners. This is an emerging market, but once the threats materialize it will accelerate quickly. Selecting the design partners to pull the product in the right direction is critical in order for products to be ready to scale once the market solidifies.
- Understanding the right integrations. Some of this technology may live in existing platforms like browser, Zoom, phone devices, etc. Architecting your system for inference at massive, cost-effective scale is key.
We are actively looking to partner with founders in this space and speak to security leaders thinking through their security programs. If you are a founder or security professional working on deepfakes, please get in touch with me at jason@greylock.com. If you have any additions or suggestions to the blog post, please reach out as well.