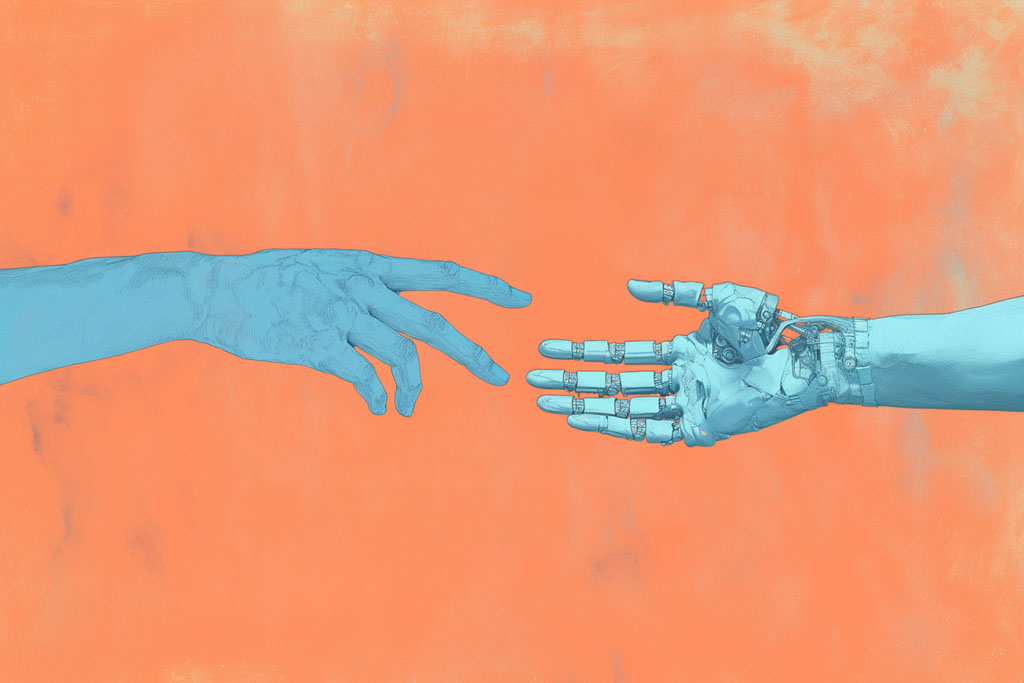
Learning from mistakes is one of the most important skills that humans possess. Ideally, this knowledge evolves over time — to the point where we can prevent or mitigate future mistakes from even occurring.
But computers evolve far faster than the human brain. In recent years, artificially intelligent systems have progressed at a breakneck speed to serve critical data insights. But it is much harder to program the nuances of emotion, societal context and overall ethical considerations that humans learn from their biggest mistakes. Too often, the unintended negative impacts of AI – such as racial, gender or socioeconomic bias in AI tools for hiring, housing, or legal assistance – aren’t understood until after its implementation.
If AI is to serve the collective needs of humanity, how should machine intelligence be built and designed so that it can understand human language, feelings, intentions and behaviors, and interact with nuance and in multiple dimensions?
It starts before the technology development even gets underway, says Stanford University computer science professor Dr. Fei-Fei Li, who is the co-founder and co-director of the Stanford Institute for Human-Centered AI. The organization works to advance AI research, education, policy and practice to improve the human condition. In June, HAI launched the Ethics and Society Review Board. The program, which was piloted over the last year, requires AI researchers to consider the ethics of their projects before they can receive funding from HAI.
“Fundamentally, how we create this technology, how we use this technology, how we continue to innovate – but also put the right guardrails – is up to us humans doing it for humans.” says Dr. Li, who also served as director of Stanford’s AI Lab and previously worked as VP at Google and chief scientist of AI/ML at Google Cloud during her Stanford sabbatical in 2017 through 2018.
Dr. Li recently joined Greylock general partner Reid Hoffman on Iconversations to discuss the ethical considerations researchers, technologists and policymakers should make when developing and deploying AI.
Throughout the discussion, Dr. Li and Hoffman explored the extensive AI landscape of today, including the practical applications for AI; how academia and industry leaders are working with the federal government to advance AI research; and Dr. Li’s work as the co-founder and chairperson of the board of the national nonprofit AI4ALL.
You can listen to the conversation by clicking on the link below, and you can watch a video of the conversation here.
Episode Transcript
Reid Hoffman:
Thank you all for joining us for today’s Iconversations. It’s my pleasure to introduce my friend, Dr. Fei-Fei Li. She is the Sequoia Professor of computer science at Stanford University and the Denning co-director of the Stanford Institute of Human-Centered AI, also known as HAI.
Before founding HAI in 2019, she served as the director of Stanford’s AI Lab. She was a VP at Google and chief scientist of AI/ML at Google Cloud during her Stanford sabbatical in 2017 through 2018.
She is also a co-founder and chairperson of the board of the national nonprofit called AI4ALL, focusing on training diverse K-12 students of underprivileged communities to become tomorrow’s AIs leaders. Obviously, we all know that’s super important, and thank you.
Among her many distinctions, she is an elected member of the National Academy of Engineering, the National Academy of Medicine, and the Academy of Arts and Sciences.
Dr. Li also serves on the 12-person National AI Resource Task Force commissioned by the Congress and White House official Office of Science and Technology Policy, which is super important for all of us, so thank you.
So let’s get started. Fei Fei, it’s been more than two years since you started the Stanford Institute of Human-Centered AI, or HAI as we call it. What’s the goal of the Institute and what have you accomplished so far?
Fei-Fei Li:
Yeah. First of all, Reid, thank you for the invitation and as always, it’s such a pleasure to just have a conversation with you.
Yes, HAI has been two years old, half of which is during a global pandemic, but we were born out of a very important mission. We want to advance AI research, education, outreach, and practice, including policy to better human conditions, because we believe this is such important technology. It’s one of those revolutionary horizontal technologies that will fundamentally change the way businesses conduct themselves and people live their lives, so we want to be focusing on benevolent usage and purpose of this technology.
So what has happened? Well, there’s a lot. Let me just try to be brief since our focus of our work is in research, education, and policy.
On the research side, we have more than 250 faculty and hundreds of student researchers involved in all kinds of interdisciplinary cutting edge AI-related research. Thanks to our generous friends, we have multiple programs encouraging everything from moonshot projects to seed level budding ideas that include AI for drug discovery, AI for poverty assessment, AI for future of work, fundamental reinforcement, learning algorithms, everything, spending tons of dozens and dozens of disciplines.
On the education side, HAI focuses on both educating our students as well as the community and the ecosystem. Within Stanford we have encouraged and continue to support multiple courses.
Some of the courses are really new. For example, technology and ethics has quickly become one of the most popular undergraduate and graduate-level classes. On campus we have courses on AI for human wellbeing and AI for climate, AI for healthcare focusing on data and fairness and all kinds of education programs.
Externally-facing, we recognize the responsibility of Stanford and our AI expertise. We particularly recognize the lack of opportunity for getting objective information about AI. So we focused on working with, let’s say, policymakers, congressional staffers to train our nation’s policy makers. We also have courses towards business executives and we have courses towards reporters and journalists. And we’ll continue to expand that external education program.
Last but not the least, we believe in this era of AI and technology, it is so important that we can provide a platform to work with policy makers at both the national, international as well as state level. As you mentioned earlier, I’m personally honored to be on a task force charter by the Congress for National AI Research Resource, but we are working with multiple federal agencies and policy makers on various aspects of AI. So that’s a short summary of what HAI is busy doing.
RH:
Well, and obviously I’m familiar since I’m chairing your advisory board with the feds.
So we’re trying to get it for everyone who is joining us to get some understanding, but what they should have heard already from your description is how much HAI is saying, We have this focus on what is good for humanity, and then, How do we build lots of important bridges? Bridges to the policy world, bridges to the research and academic world.
One of the other important bridges, which I think will be particularly useful to this audience, is what’s the role of the institute with respect to industry? What are the kinds of the interactions and the kind of the thing that industrialists or technologists or the industry should look at HAI and think?
FFL:
Yeah, Reid, great question. First of all, let’s just recognize this:
“First of all, let’s just recognize (in the AI age) that industry is one of the most vibrant and fertile grounds for both innovative AI applications as well as career AI research.”
So it’s such an important part of the ecosystem. And frankly, I think it’s such a unique strength of America for the past decades, if not a century.
At HAI like the entire Stanford community, we fiercely and profoundly believe in our academic freedom and independence. In fact, that value statement is on our very website.
Having said that, we also believe in a lot of free exchanges and ideas and forums for discussions.
So from that point of view, HAI is actively engaging with industry partners. For example, to begin with more formally, we have industry partnership as corporate partners and affiliate programs where we can engage in research exchanges and ideas. Of course, this is protected under our academic freedom and independence as a policy. But more than that, we see ourselves at Stanford to be a rare platform where industry partners, colleagues, civil society, policy makers, researchers of all disciplines can use it as a neutral platform to discuss, debate, and explore ideas of frankly, some of the toughest issues of AI.
Just give you an example, Reid, I know you know this, we geek out on this, GAN: generative adversarial network. This is a multiple of a name for a really exciting neural network technology that can generate images, speeches, texts. Of course it can be used for creative usages for generating training data.
These are all great uses, but it’s the same technology that can be used for fake disinformation. And how do we continue to exploit this technology for better use, but put guardrails? These are tough questions – and industry innovators and entrepreneurs are trying to use this – but policy makers, civil society, stakeholders are thinking of the guardrails.
Stanford provided a platform for them to get together and discuss this. Another example is for recognition technology. This is a technology compared to many other AI technologies to a certain degree of maturity, yet it also can cause a lot of harm from bias to say, surveillance.
And how do we really grapple with these challenging issues? We continue to provide forums and platforms for industry leaders and partners as well as other stakeholders to come together and discuss this. Absolutely we see the value of our ecosystem and industry is a huge player we love to continue engaging.
RH:
As you described, it’s super important for industry because it gives an independent, motivated by truth and integrity and objectivity that is in the academic side to build bridges, but also to give good feedback and good ideas into industry.
Too often, especially the technology industry thinks it can get, Ok, we’re good. We’ll just do it alone. And it’s like, No, no, no. This is getting too important. And part of that “too important” is that AI is obviously going to redefine many of the landscapes of industry and therefore have really serious impacts on society.
And I think it was your call to arms in a New York Times article, a short essay that you published about putting humans at the center of AI, and therefore the name of the institute obviously. Tell us a little about how you define the term and why it was so important to be human-centered.
FFL:
Yeah. Thank you, Reid. Yes. So I always believe that since the dawn of human civilization there’s something in our species DNA that we will never stop innovating. We innovate all kinds of tools to better our lives, better our productivity and to frankly also interact and change our environment.
But these tools are fundamentally part of human creation and part of the human fabric. So now that we don’t call them tools, we tend to call them machines because they’re much more sophisticated. So philosophically I do believe that there are no independent machine values. Machine values reflect our human values.
“AI is exciting. This technology is made by people and it’s going to be used for people. Fundamentally, how we create this technology, how we use this technology, how we continue to innovate – but also put the right guardrails – is up to us humans doing it for humans.”
So at the heart of all this, it’s all human-centered. And that’s how I see this in a fundamental way.
And of course, Reid, I hope it continues to enhance our humanity and capability and impact our human community, human lives, human society in a benevolent and positive way.
RH:
And thinking about the human side, let’s take it a step more personal. What was it in your early career that prompted you to focus on the human side of AI? It’s unusual for someone who is as deep in computer science and engineering and technical excellence as you are.
FFL:
So, Reid, here’s a secret. I don’t think I ever said that. I don’t have a computer science degree. My journey into all this started from physics. I was deeply, deeply (just like you) asking those fundamental questions of the beginning of the universe and then, What is the smallest particle or structure of the atoms?
And that love for fundamental questions led me to the writings of the 20th century physics giants, like Einstein, Schrodinger, Roger Penrose who just got the Nobel Prize by the way last year. And I noticed that these physicists in the second half of their life start asking a different kind of fundamental question. And it’s the question about life.
And that led me into a… I guess now it’s a lifelong passion towards trying to understand the fundamental questions of life. Questions that really captured my imagination, even in my early undergraduate years, were [about] intelligence. What makes intelligence arise in animals and especially high intelligence in humans?
And so I started my entire journey in intelligence with human intelligence, human neuroscience, human cognitive science. But I guess still thanks to my physics background, I quickly gravitated to the mathematical principles of what is the underlying mathematical expression of intelligence, and that got me into computer science. So it was a very long journey, but along the way I had unusual training as well as exposure to human neuroscience, cognitive science.
And one more dimension to the human side of this technology is also a personal journey. I happen to come from a fairly humble background as an immigrant. As an entrepreneur, I opened a dry cleaner shop and ran it for seven years. I have a parent whose health condition is fairly weak. So I had a lot of interaction as just a person living a life where I see how human lives can be impacted by incredible technologies.
And so this part of the philosophically intriguing quest for intelligence, plus the grounding human life that I experienced on a daily basis, [that] continues to point me to the belief that technology can be framed in a human-centered way, science and technology. And we can seize every opportunity we can to make it human benevolent.
RH:
Yeah. And actually obviously the personal side naturally leads into the starting of some questions around industry, because obviously you’ve participated in industry in multiple ways, not just the putting your school and supporting your family through dry cleaner, but actually in fact the major functions at Google Cloud and others.
So what are you personally excited about with the role of industry in AI, and then which industries most benefit from applied AI, and then obviously the thread of how human-centered AI plays into that?
FFL:
Oh, yeah. Thoughtfully, Reid, you and I talk about this. I think industry, I’m tremendously excited. I actually think the democratization of this technology, the innovation, and eventually the human impact of this technology is mostly delivered through industry, through startups, through companies, through their products and services. There’s no doubt about it. And I was very thankful to have that sabbatical experience at Google and seeing that.
Because at Google Cloud, we serve enterprise businesses, we see different vertical industries, right from healthcare to financial institute, to energy gas, to media, to retail, transportation, you name it.
So I’m just very excited. And also I’m very, very excited just like you of these budding, our new entrepreneurial efforts, the startups. Because AI is very new. The sky is really the limit in terms of how we imagine this technology can serve human wellbeing.
And personally, there’s definitely one industry that I feel deeply, deeply connected to through my research and personal experiences, healthcare. 10 years ago I was still directing Stanford’s AI lab. And, Reid, you remember ten years ago was really the Silicon Valley when the world was in the middle of the excitement for self-driving cars. Because of convergence of technology, the sensors, the algorithms are the hardware of course and maps. Technology is leading to this realization. Transportation and mobility can be re-imagined.
And during that time, it really dawned on me: Perhaps during one of those hospital stays of my mom that I realized that a similar way of using technology can be applied in healthcare industry, where one of the major pain points of our patients and clients is all the lack of context of what’s happening to the human in the center of this, and that human is the vulnerable patient.
My mom is a cardio patient. Doctors constantly want to know how her behavior is, how her heart rate is changing because of the activities. And also in the hospitals, doctors and nurses worry about patients falling, having accidents, and pulling their IV lines. All of these things are the lack of knowledge, lack of context of patient behavior.
So I started this program at Stanford with Dr. Arnie Milstein on what we call Illuminating the Dark Spaces of Healthcare – the MBA intelligence of healthcare, and started researching on how AI sensors edge compute, deep learning algorithms of human behaviors can help doctors and nurses and patients to recover better, detect conditions earlier and keep them safe.
And then I continue to work on this at Stanford, and I continue to feel very excited to start to see that there are startups starting to get to the space, innovating rapidly in this space. And I really want to see one day I don’t have to worry about my mom if I’m at work or not with her and her health wellbeing is being helped by AI technology.
RH:
No, indeed. And actually this is a good place to ask one of the audience questions we’ve got so far, because obviously the huge opportunity in AI for health and how to transform that industry. But also one of the key questions that is frequently asked about AI models is the model safety and reliability.
So the question from the audience was sharing HAI’s effort on model safety and reliability with industry applications. Obviously it isn’t just health. Classically people think about this in the criminal justice system or the financial system, racial and other forms of social equity. But what is HAI doing and catalyzing with industry on model safety and reliability?
FFL:
That’s a great question. Thank you for asking and, Reid, I know you care a lot about this. We talk a lot about this as well.
So the word “safety” is actually loaded with different dimensions. Let me try to unpack that a little bit. You mentioned and the question mentioned fairness, which was… The flip side of that is bias, is one big chunk of safety. I’ll address a little bit. There are also other aspects including the robustness of the technology. How do we quantifiably and reliably understand robustness? There is also the trustworthiness, which has a lot to do with transparency and explainability of the technology.
And then there’s also the whole practice of how ethics can be incorporated into the design and development. So there are several buckets.
Let’s just start with fairness and bias. AI as a technology is a system. It’s the pipeline of the system starting from defining the problem to curating the data, to designing the algorithm, to developing the product, to delivering the service. Along every point of this pipeline there is opportunity to introduce bias. At the end of the day, a lot of bias or maybe all of the bias is rooted in human bias. Our history, our human psychology is where the biases start.
So I think at HAI you can see our researchers are working on every point of this pipeline, inspired. We’ve got researchers, myself included working on the upstream data bias, how we become vigilant and mitigate the bias that’s introduced into the data and how we try to fix that.
Classic example: We’ve got researchers showing that in America most of medical AI research data come from three coastal states, Massachusetts, New York, and California. Imagine while this is a good thing, we’ve got medical data to do research, it’s also a deeply, deeply biased way of using data. So we need to be vigilant and mitigate that. Then we get into an algorithm that we can all throw our hands in the air and say, “Well, the bias comes from data. What can I do?”
For example, historically, let’s say… You’re linked to LinkedIn, you’re looking at job applicants. And there are just fewer women in, let’s say, in computer science disciplines historically. But if we throw our hands in the air and say, “Well, we’ll just use whatever historical data to whatever..” it’ll fundamentally be unfair to women of today and women of tomorrow. So our algorithm, whether it’s through a different way of looking at objective functions and other more technical methods, we need to mitigate that.
And then it comes down to decision-making inference. There’s another whole bucket of technology that our researchers are exploring. I just use those to illustrate even on the bias side we have multiple kinds of research.
One other thing that I’m actually really excited about is what we call machine bias. In fact, machines are the best to call out human bias because there’s so much human bias in our data. My favorite, for example, was a few years ago: a face recognition algorithm called out Hollywood’s bias on using male actors a lot more. They have a lot more screen time and talking time than female actors. These mass data analysis and machines calling out bias is really important. And we continue to do that.
And then there is explainability and robustness research. We have researchers in medical school, in the computer science department, in gender studies programs, they are working closely together in trying to look at these robustness and explainability technologies. And of course there’s the whole design process. Reid, I know you are one of the staunchest supporters that we have. Stanford HAI has led to an innovative research proposal review process called the ethics and society review. That is a step up from the classic human subject review in universities called IRB.
But in this, what we call ESR process, HAI-funded research needs too. Every one of them go through an ethics and society review before we provide funding to support this research. And the philosophy behind this is to bake ethics into the design of the research program, not as an afterthought for mitigation.
So that was a long answer to this very profound question of how HAI, our research and our own practice is addressing this issue of safety and trustworthiness.
RH:
No, it’s a super important topic, and I’m glad you were comprehensive because it shows how much work HAI is actually doing on this topic.
I think it’s worth double clicking on the ethics and society review. What have been some of the learnings of doing it so far?
FFL:
Yeah. Good question. So, Reid, in fact you probably are aware, I’m also aware, even companies are now trying to practice ethics review for their products. I think what is in common is everybody recognize the importance, but what is special (and I take a lot of pride in this) is that at Stanford we have true experts from sociology, ethics, political science, computer science, bioethics, law coming together to form that deeply, deeply knowledgeable panel and their job is to help our researchers. Many of them are maybe just deeply technical researchers that do not have the training to guide them to think about when they design their project. What are the human ethical societal impacts that might come out of this research intended or unintended?
I’ll use a personal example because that’s closer to my heart. I talked about our healthcare research in AI that uses smart sensors for example, to help monitor patients who are at the risk of falling in a fragile seniors home. And that’s a very painful problem in America. More than $40 billion of healthcare money is spent in mitigating potential falls for our seniors and every fall costs lives, pain, quality of life, but it costs a lot of money. But as we are excited as technologists to think about how computer vision and smart sensors and edge computing can help, we were also confronted with the question of privacy, with the question of legal ramification that we never thought of. What if the sensor picked up care abuse cases? Can they serve as legal witnesses or some other adversarial events?
We also have not thought deeply at the beginning of how to explain, What’s the interpretability of this technology? Especially for a caretaker like an adult child trying to decide for their elderly parents if this technology is good for them.
And as we write up our proposal, as we go through this ESR reveal process, the bioethicists, legal scholars, and philosophers formed a panel to start to guide us towards how to think about this. For example, one thing I think that came out that was so cool was that the privacy concern pushed our technology further. It pushed us to think about all kinds of secure computing, federated learning, more modern encryption.
“I think some always fear that guardrails slow down innovation. In many cases, I disagree. I think these kinds of human ethical concerns push our technology further.”
That’s one personal experience in this research.
One thing that is really fun we have learned about this process (because we’ve only beta tested this for one year) is how technologists tend to want to have more freedom, a bigger box to play. In this case, it provided so much value. When we did our survey, the engineers and scientists asked for more of ESR to the point the panel is like, “Oh my God, we need more resources to beef up our team.”
So it’s really heartening to see that there is mutual recognition. There’s no us versus them in this. We are all humans. We as technologists want the best for us as the community. And they are asking for more of this.
So we were so encouraged to see this one-year program and we’re absolutely doubling down. We’re absolutely going to continue to expand on this. We hope the whole of Stanford adopts this program.
RH:
Well, and the world more generally.
The whole goal of innovation is the right innovation, the right outcome. And so when you say, “Hey, ethics is important.” It’s like, “Oh, that just slows us down.” No, that helps us accelerate towards the right outcomes, is the key thing. And then, actually, when folks are engaged with it, they find it productive and useful and energizing.
And so this is one of the things that industry people can learn as well in this, because, in fact, this isn’t going to be the, Oh, it’s bureaucracy. Oh, it slows me down. It’s No, no, no: It’s accelerating you towards the right outcome, and then feeling the mission and the energy in your blood, in your heart about where you’re going. And I think that what you guys have been doing with ESR is important and everyone should know. That was the reason I doubled down on that question.
FFL:
Thank you, Reid. And also frankly, Reid, I believe that is a business competitive advantage. When you make more trustworthy and safe products and services, you’re better off in your market. So it really is not to slow you down or to put you in a competitive disadvantage. It’s quite the opposite.
RH:
Yes. Exactly. And so one of the things obviously that people… When they’re confronted with new technology – and as a part of what we’re seeing in society and concerns around AI and privacy and data, and a bunch of things – they worry a lot about the negatives, and it’s important to pay attention.
That’s part of the reason why the model safety ESR, but one of the things I fear is too often lost in this is the amazing upsides. The fact is No, no, no, we’re playing for greatness. We’re playing for something that could make huge differences in society. And that’s very important, kind of classic English idiom, don’t throw the baby out with the bath water.
And so let’s return to AI in healthcare. That’s one of the areas that you’ve been personally intensely focused on in addition to all of AI and industry and policy and all the rest. Talk a little bit about the ways that you’re seeing that AI can benefit healthcare. What is the future we should be accelerating towards?
FFL:
Yeah. I mean, Reid, I know we talked a lot about this. Oh my God, healthcare is, in my opinion, the most important industry that can take advantage of AI and it is also so human-centered. It’s not just human physical wellbeing. It’s also human mental wellbeing and human dignity. And it frankly does excite me to work in an industry where the benevolence is so pronounced and it’s the goal of the industry.
So one thing about healthcare that’s really paradoxical, Reid, is it’s actually extremely data rich. So one would think if it’s data rich, it’s AI rich, but it’s not true. It’s data rich and insight poor. You can stick the patient into all kinds of imaging labs and all of that and suddenly the result is that your clinicians and doctors, nurses are overwhelmed, overworked, overcharting, and spending too much time charting and yet they don’t have tools. They don’t have opportunities to glean important insights from what’s going on in the patient.
So I absolutely see this is a huge area of opportunity for entrepreneurs and startups and companies to really focus on – not giving our doctors and nurses more overwhelming amounts of data, but it’s really how we deliver critical insights that is timely and precise and accurate to really help our patients. And that’s one huge area.
The other area is absolutely decision support, and also just productivity support. I lived with my mom in hospital systems for 30 years. Every time I go, I see the nurses and doctors overworked. An average shift a nurse works 200 plus tasks, walks five or four miles per day, and spends two hours charting. And the American nurses burnout rate is outrageous. 33 of our nurses leave the job after two years.
These are unacceptable. The heart of healthcare is humans caring for humans, yet our clinicians are not spending time with the patients. And anything this technology can do to reduce that burden, to support their work, their productivity at the end of it is their humanity will help our patients.
So that’s another area of opportunity in healthcare. And of course drug discovery, we’re just at the beginning. Reid, you’re probably even more on top of this. The past year I think there’s been a huge heating up of drug discovery investment and startups. And this is big thanks to a lot of these molecular cellular genetic technology, but they are turning out volumes of data.
And now with machine learning, it can help glean the data and help discover important drugs. Radiology is a classic example of machine learning support. Even public health that the global pandemic has taught us that we extremely have a data issue. We need to break the barriers of data. We need to modernize the way public health data is organized and information can be gleaned.
One thing, Reid, I want to finish by emphasizing in this question is this: AI as well as the surrounding technology is what I see that can augment the humanity of the healthcare industry, not to replace. We’ve heard of people talking about doctors being replaced and nurses being replaced. As someone who spent 30 years as a patient family, I can tell you, no one can replace them. The human-to-human care, human intelligence and emotion is critically at the very heart of this industry, but anything AI can do to enhance that is what I see as exciting. And the opportunities are boundless. I’m just super excited by this.
RH:
I completely share that with you. And actually let’s generalize from that last part, because it’s not just not replacing nurses, not replacing doctors, but actually in fact part of human-centered AI is to amplify the ability to work well, work meaningfully. And one of the common misconceptions about AI is it’s going to replace jobs and people, and look to some jobs that will be made so much more efficient. There may be fewer people in them, but generally speaking, what we find is that AI can help collaborate with people and help productivity.
And we have Erik Brynjolfsson and his lab at HAI as part of one of the things that you and Erik and Andy put a lot of energy into making happen. And I also know that you’ve been shifting some of your research to robotics, because AI is obviously going to be central to robotics. What do you see happening with robotics in the business world, and what do you see about that and human work?
FFL:
So first of all, healthcare is my application area, but my foundational research now is more in robotics. Let me just say to you that I’m so excited intellectually by robotics because that is the closing the loop of nature. That a living, moving, interactive organism – through the course of hundreds of millions of years of evolution – can lead to an organism like humans, is nature showing us that intelligence and action come together to brew together this incredible machinery.
And robotics research is a vehicle to that. You suddenly have a system that can perceive, can learn and can do. And that is the future of AI. So whatever revolution we’ve seen in the past 10 years, Reid, I think it’s a prelude of what’s to come, what’s more exciting to come.
And in that sense I definitely have shifted from passive visual intelligence in computer vision to the more active robotic perceptual robots research, but that also has a profound impacting industry. In fact, I mean, obviously manufacturing, but there is fulfillment, agriculture. Everywhere humans are conducting a lot of physical labor, robotics is potentially an area that can become assistive technology.
And in fact, to start with, I actually do believe there are certain types of work that humans need to be replaced by machines, especially the work that puts humans in danger, whether it’s deep water exploration or a lot of rescue situations or other dangerous work. But you and I have talked about it.
Our friends at McKinsey have told us repeatedly it’s the tasks that might be replaced and assisted, not necessarily the jobs. Almost every human job consists of multidimensional tasks – many, many different kinds of tasks. There are tasks that are difficult for humans, are dangerous for humans, and I can see robotics play a huge role.
But there are tasks that are more reserved to human cognition, human emotion and there, I just don’t see that and especially if as a society we make sure how to address these issues. So the future of work in the age of AI is a profound question. It inevitably will impact workers, but the collective efforts in how we train the future workers, how we mitigate skillset shifts, how we address job landscape evolution together with how we use technology in the smart and humane way. I’m hopeful that humanity, having gone through several rounds of industrialization and labor shifts, can address this together, but we have to be mindful of how we do that.
RH:
There are a lot of countries that are engaging in AI, and you were recently appointed by the White House to the National Artificial Intelligence Research Resource Task Force. This task force launched due to efforts HAI led to call for a national research cloud – super important – which resulted in legislation passed in January to create the task force and make recommendations. Awesome.
So what role does HAI make in making America more competitive in AI? And then, how are you helping the government (and the government as it interfaces with industry) understand the risk rewards for the future for AI?
FFL:
Yeah, important question, Reid. First of all, as we discussed earlier, America has been very unique. We have the world’s healthiest, most vibrant ecosystem for the past, more than half a century, closer to a century in terms of innovation. And our innovation goes as upstream as basic science technology all the way to the practice and the industrialization, commercialization of our technology. And that ecosystem brought this very prosperous society for us.
Of course, it’s an imperfect society. We have a lot of issues to address from the way we look at how different groups of people are treated and meaning imperfections, but it’s a society that’s rooted in the belief of democracy, the belief of human rights and human values, the belief of equality and justice.
And I think that combination of such a healthy vibrant innovative science and technology ecosystem plus the country’s value is really important to all of us. And so is it important to HAI.
So to start with, we hope to be a player and to contribute to that ecosystem. Academia is where some of the most innovative science and technology happened, including deep learning. It first happened in academia. So we want to continue to contribute, but we also want to continue to support policy makers to support America’s ecosystem.
This is why we participated in the legislation. This is why I’m personally honored to be part of that effort. So needless to say, we see ourselves as a player. We stand by to help our nation and to rise to the occasion whenever that’s needed. And most importantly, we educate our nation’s future and we will continue to do that.
RH:
Yeah. And speaking for many, many of us in industry technology, thank you for your public service on this. It’s really important to the nation. You just spoke so eloquently about America, the idea, its values, its aspirations.
So I think it’s fitting that this will get to our last question, which is [about] increasing diversity in AI. And because part of the thing about the future that we want to build is to make sure it’s inclusive for all of us. And one of the things that you founded, and – in addition to public service – put a lot of personal energy and time into, is AI4ALL. So if you could say a little bit about that and then also how people can help with it.
FFL:
Yeah. Thank you, Reid. So talking about America, one of the most beautiful thing about America is we’re a nation of all people, people of all backgrounds, people of all race, people of all walks of life, but it’s also a reality in today’s world, in our country, for example, in the AI world, it’s not well-represented. We’re lacking women. We’re lacking people of color. We’re lacking people of all walks of life.
And I realized that it became really front and center to me as the deep learning revolution was taking off around 2014. And my co-founder, former student Olga Russakovsky and I recognize this really important question, is that if we know and believe AI will change the world, change our future, the key question is really who will change AI? Who would be at the steering wheel of designing, developing, and deploying AI?
Once we asked that question, we realized we knew the answer. We don’t know how to reach the answer – oh, we have a long way to reach the answer yet! The answer is we want the representation of the world, of America, to be at the steering wheel of AI. That means we want to invite a lot more students from underrepresented, underserved backgrounds who were not traditionally part of this technology to be trained as tomorrow’s leader.
That was the birth of the national nonprofit AI4All focusing on K-12 education of AI. And we serve high school students who will come to different chapters of AI4ALL across the nation, (around 20 of them, but still increasing), to learn about AI in our summer programs. We partner with local universities and colleges so that the education of these students is tailored towards the community needs.
We also have an online program to encourage both teachers, K-12 teachers and students to participate in understanding AI. And we also have a couple of programs geared towards our alumni throughout their college years and early career to mentor them into the workforce of AI and want to make sure they become tomorrow’s AI leaders. So AI4ALL is a growing national nonprofit organization. We partner with companies. We partner with mentors who believe in this mission. And we of course partner with supporters who believe in our mission and we would love to work with any of you out there who would believe in us and help us.
RH:
So, Fei-Fei, an honor and a pleasure. Thank you.
FFL:
Of course, the feeling is mutual. Thank you, Reid. Always great to have a conversation with you. Have a great day, everybody.