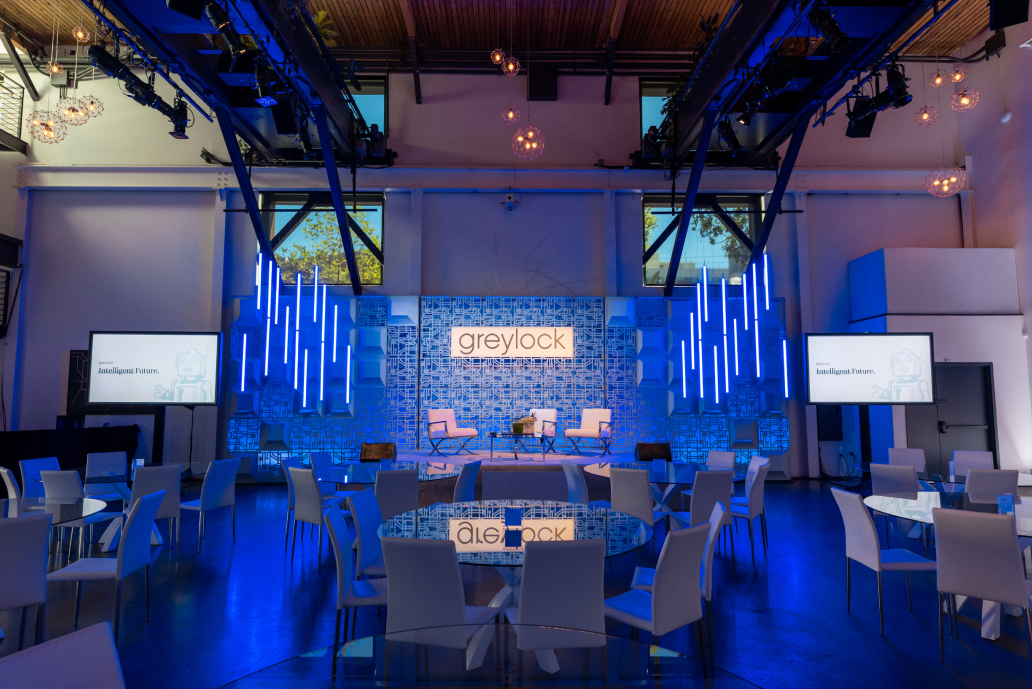
The pace of advancement in artificial intelligence technology has rapidly increased in recent years. That has led to an explosion of new companies and products aimed at amplifying and extending human efforts both in enterprise and consumer markets.
Listen to this article >
This is evident both in the evolving everyday software stack – which includes products such as advanced data indexing and management systems, and applied machine learning tools that automatically counter cyberthreats – to the emerging use of large language models that are able to generate sophisticated artistic and linguistic creations. Each development has spawned a wave of innovation that has opened up countless opportunities for large, established businesses and fledgling startups alike.
As we live through this moment of profound advancement, we are also tasked with identifying the many potential risks associated with AI, and in turn, making many ethical considerations when developing tools.
To gain a more cohesive perspective of the industry, we recently hosted the Intelligent Future: a day-long summit with AI experts, visionaries, and early stage innovators across the field. Guests include Microsoft CTO Kevin Scott, OpenAI CEO Sam Altman, Stanford professor Dr. Fei-Fei Li, and founders from some of the most promising AI startups today. In the coming weeks, we will be sharing the conversations from that day on the Greymatter podcast and our YouTube channel.
To kick things off, we sat down on the Greymatter podcast to talk with Blitzscaling co-author Chris Yeh about everything we are seeing in the industry, and to set the stage for the subsequent discussions with our guests from the summit. You can listen to the interview at the link below or wherever you get your podcasts.
Episode Transcript
Chris Yeh:
Hello, and welcome to Greymatter. The podcast from Greylock. I’m Chris Yeh and I’m here with Greylock General Partners, Reid Hoffman, and Saam Motamedi. Today, we’re going to talk about artificial intelligence.
Now, Greylock has partnered with quite a number of startups in this rapidly advancing field, including Abnormal AI, Inflection, Adept, Snorkel, Neuro And Cresta. Reid and Saam have led many of these investments and have a unique vantage point on developments in AI.
This discussion is a precursor to a series of conversations Reid and Saam will be having with top experts, visionaries, and early stage innovators across the AI ecosystem. They’ll discuss everything from the latest achievements in robotics and applied machine learning, to large language models, and the ethical considerations of AI. And of course, they’ll explore the many new opportunities for startups within this field.
Guests of this series will include Microsoft CTO, Kevin Scott, Stanford professor, Dr. Fei-Fei Li, OpenAI CEO Sam Altman, and the founders of some of the most promising AI startups today. All of these conversations will be available in the coming weeks on the Greymatter Podcast.
So, gentlemen, let’s start with some of the basics. What are some of the biggest technological developments in AI in recent years? And what do they mean for the state of the field?
Reid Hoffman:
Well, one of the things that’s pretty interesting here, Chris, is that there’ve actually been a number of really important ones. Like the whole artificial intelligence field is a renaissance. My undergraduate major was symbolic systems at Stanford, which was kind of symbolic intelligence and cognitive science. And while some of those same techniques are used in deep learning and neural networks and that kind of thing, there’s also been a huge number of new things.
And so even though I’m going to throw out a few, it’s just beginning. So one of the really central things was kind of creating a new set of paradigms for training these models. Generally speaking, multi-billion parameters, kind of can be 20, can be 250, going on 500, et cetera, kind of large network models. One part of the training paradigm is this thing called transformers, which is, roughly, take a lot of data and text and then start predicting, using the removal of interesting words, start predicting, and that becomes a generative model.
And that kind of generativity then creates all kinds of interesting possibilities, not just a generational language, but a bunch of other things. But by the way, part of the reason I start with training paradigms is kind of goes all the way back to some of the Deep Mind stuff that Demmis, Shane and Mustafa Suleyman did, which is like game on game. Training it by playing itself in AlphaGo or chess and other kinds of, or pong, and ways of doing things. So there’s a whole bunch of supervised and partially unsupervised and unsupervised training paradigms.
This leads to large language models and foundational models, which is where a lot of the magic tends to, the most amazing magic currently tends to come from. And then there’s image generators, which use diffusion and other kinds of models too, with most notably with OpenAI’s DALL-E, that create pictures that even when you say kind of like, “Okay, a future city in watercolor,” it will actually give you a watercolor image of a Jetsons kind of future and so forth.
I recently did some essays on this and kind of released some NFTs. Because another thing we do at Greylock here is also look at the Web 3 stuff. And so it’s kind of putting these together as part of it. And those are just, I mean, part of what’s amazing is those are some highlights, but that’s just the beginning.
Saam Motamedi:
Yeah. And just to tack on, I mean, Reid called out a couple of the really important trends here. One of the things that’s been interesting to me is just the focus and innovation we’ve seen around generative. I think if you rewind several years ago, a lot of the focus was on discriminative models. So show me a photo and tell me, “Is this a cat or a dog?” And that’s interesting, but we talked about DALL-E on the image generation side, we’ve seen models like GPT-3 on the text generation side.
And what’s interesting is we’re now beginning to see the applications of generative actually go mainstream. So today, if you’re a copywriter, you can have AI help you actually dramatically increase the speed at which you develop copy, whether that’s marketing copy, writing a job spec. We’re going to see a lot of really interesting things get built on top of a mixed generation and models like DALL-E. And so I think generative is a really, really important area.
The second thing I’d call out is kind of the combination of these large language models with program synthesis. And the ability to take action on behalf of users, I think, is something that we’re beginning to see early signs of. And we’re going to see more and more of. So imagine you have models that can understand, but then actually act on behalf of the end user. And in an enterprise context, take actions in business applications to kind of automate workflows. I think that’s going to be important.
And then a third piece is just what’s happening on the infrastructure side. So if you think about the pipeline to actually building a machine learning application, starting at data management, and then doing things like model discovery, training, serving, monitoring, there has been a lot that’s happened over the last 18 to 24 months – both in terms of new companies and open source projects – that make each step of the pipeline much, much easier.
And so for new application developers, new product builders, there’s a lot to actually leverage and get your AI products to market much faster. And so I think these are all ingredients that are going to lead to a complete explosion in the number of AI applications we see over the coming quarters and years.
CY:
I’m struck, Saam, by your notion of this generativity and activity on behalf of the user. That really seems like it’s fundamentally different from what came before. It feels almost like, to draw an analogy from earlier, the transition from web 1.0, to web 2.0. Things just open up and there’s just so much more that’s possible as a result.
“For new application developers and product builders, there’s a lot to actually leverage and get your AI products to market much faster. These are all ingredients that are going to lead to a complete explosion in the number of AI applications we see over the coming quarters and years.”
SM:
Yeah. And one of the things that’s interesting is in some ways, it’s almost easier when you have your product hat on. Because a lot of these generative products can build in ways where a human’s in the loop. So I can use the generative text model and say, “Hey, I’m trying to build a job description for a software engineer.” And the model can take me 90% of the way there. And then I can come in and do that last 10% of tweaking.
When we think about backing new founders building AI, we both think about what’s possible from a technology perspective, but then also what areas lend themselves to products that can actually get to the accuracy and effectiveness that end users need to really transform the way they work.
If you take that lens, I’m really excited about generative because I think it lends itself really nicely to these human in the loop workflows. Where the model can do the heavy lift, the end user and specialists can come in and do a little bit of fine tuning if you will. It’s an overloaded term here. And then get to something that’s actually very, very performant.
CY:
Well, I’m very glad to hear that the human remains in the loop because us authors have a lot to fear if generativity just takes over. But I’m glad to hear I’m in the loop for just a little bit longer.
SM:
Absolutely.
RH:
This is actually an important point to kind of emphasize. Actually, in fact, people tend to jump too quickly (especially within Silicon Valley), and go, “Okay, these products are on a performance curve and improvement curve, so, therefore, soon humans will be out of the loop.”
And actually in fact, it’s not to say that it’s not impossible in various contexts. But when you look at GPT-3, when you look at DALL-E, when you look at the music generators, and all these things, if you actually say that, you shift your paradigm some into thinking about how these tools now become the essential tool. For example, you say, “Well, I’m a writer.” And if you kind of don’t write using computers these days, you’re actually very unproductive.
But you say, “I got my typewriter, or I got my pen and paper.” It’s like, no, no, actually in fact, the computer is useful in all kinds of ways. Well, this is like that amplification of that. Similar, like I’m a graphic designer or an artist. It’s like, well, it’s a tool that’s like Figma or like Adobe. And so it isn’t that the generativity suddenly goes now, Chris, instead of writing, you can sit back and eat popcorn and watch the movie while GPT-3 is writing for you.
It becomes a thing that is an essential part of your toolset for how you generate quicker, better, faster at a higher quality output for all of that. And it just becomes an essential tool, just like we, as creative professionals, have these essential tools now. It’s like, for example, you say, “I’m going to create a movie, but I’m going to use it by snapping Kodak Polaroids.” It’s like, well, that doesn’t work. Let alone, not even as photography. And so these are just now the essential tools by which this is going to operate.
SM:
I remember at Greylock several years ago, we were discussing these things. And there was a lot of skepticism in the kind of different experts and different people in AI we spoke with. And one of the things that I think everyone has changed their perspective on is that we’re not hitting the training models on scale. And so that’s what’s so interesting is, if you look at these models, and as we increase the scale of data and training runs, they just keep getting better and better. And that combined with their ability and some of the mechanisms that have been unlocked, including the ability to train on different modalities of data, again, really changes the types of, to use Reid’s parlance, magic they can do. And I think we’re very early days of that. I think if we’re all talking again a year from now, we’re going to be stunned by some of what’s possible.
CY:
Well, that’s one of the things about these technologies. I mean, people try to predict them and they always predict them in a linear way. Whereas actually we live in an exponential discontinuous world, and the stuff that comes is never the stuff that people predicted.
RH:
Part of the thing that I think is amazing about technology is that usually the progress is sooner and stranger than you think. So, like in the 1950s people thought of flying cars because they had cars and they had airplanes. Like, okay, flying cars. Now we’re just beginning to get the flying cars. Joby, other kinds of things, as a way of doing this. But what did we get in the interim? Well, we got the internet, we got mobile phones. We got a whole bunch of things which were never part of the Jetsons future. I mean, sure, Star Trek had communicators et cetera, et cetera. You think the smartphone’s a whole lot more interesting than the communicator in Star Trek. Even with a little hit the little button on your vest as a way of doing it. The smartphone is more of an interesting platform.
And that kind of “sooner and stranger” is the kind of thing that we’ll be seeing that you’re gesturing at with the kind of exponential technologies or fast developing technologies. And part of what’s interesting about AI is actually we’ll be amplifying a bunch of different human capabilities. And that’s part of the thing, is amplifying these capabilities in ways that it doesn’t necessarily mean everyone tends to go to the oh, wait, is that going to replace a whole bunch of jobs?
And for example, if you can provide, call it, say a two-X amplifier in engineers, you’re not going to shrink any engineering jobs whatsoever. There is such a massive amount of headroom for it. And by the way, that’s not just true for that. That’s actually, I think true for lots of different kinds of content creation. I think it’s true for visual design and DALL-E. I think it’s true in writing and other kinds of things.
Like, well, if you give me an amplifying tool, sure. And so that’s part of where this kind of human amplification, what Saam was earlier referring to as human in the loop, will be there for a fairly long time. Even as we’re going to see this kind of magic of amplification and the kind of things being done by these AI models. That just literally, this pattern wasn’t deemed as possible or near term possible even five years ago.
“Part of the thing that I think is amazing about technology is that the progress is usually sooner and stranger than you think.”
CY:
Now it’s funny, you mentioned George Jetson. Of course the internet’s gone crazy recently because it is in fact true. If you look back over the cartoon, you can basically do the math and George Jetson was born in 2022. So we are literally living in the beginning of the Jetson age.
And what’s funny about the Jetsons is it’s this image of technology being just part of the every day. Everyone has adopted every technology, flying cars, briefcase cars, robots, you name it. Is that how consumer adoption of AI is going to proceed? Are people just going to dive into it and just start using DALL-E and GPT-3 for everything? Or is there going to be reluctance? Is it going to be areas where people are like, I don’t know?
RH:
Well, I think just like any new technologies, like for example, there are people who were slow adopters of smartphones, maybe even some people who don’t have smartphones now. There’s always the older set. The younger set takes to it like it’s water – a kind of oasis in the desert – and just does it. And that happens much more as there are now digital natives, et cetera.
But on the sufficiently large ones, everyone ultimately starts ending up adopting. And that was part of the reason why when I kind of got a chance to start playing with DALL-E and looked at it, and generated some essays on it. Generated some galleries, did the NFTs I mentioned. But part of it was, if you look at DALL-E, the very first thing, my very first day’s reaction was a misconception, which is like, okay, everyone’s going to have a B plus A minus graphic designer in their pocket.
That’s actually a misconception because there’s people who have no ability to generate visuals, they can still use DALL-E. They can use it for generating a unique holiday card, a birthday card, Valentine’s Day. Something that kind of may be an image because they can use their language and words and kind of iterate through it.
And it may not be as great or as artistic as a professional visual thinker and a professional tuner. And who knows what the way is to make something really amazing. But they’ll be able to use it for a variety of their use cases. Emojis. Consider emojis and communications. And then on the ones who have some skill, like for example a graphic designer, an interaction designer, an illustrator, et cetera. Who might be deployed in various contexts, not just even tech companies, but more broadly. Well, now they’ll have a tool by which they can move more quickly. Iterate 30 different possibilities.
And so for example, you say, “Well now when they’re generating a logo for a small business.” Where previously they might have to charge a small business $500. Now maybe they could charge a business $50. Because they say, “Okay, tell me what you want right now.” And I start typing into DALL-E. And I say, “Well, which of these do you most like? Oh, you want me to mod this one a little bit? Okay. I modify this one a little bit. Here you go.” And I can provide a bunch more.
And then I think that goes all the way up to artists. Where directors will do pre-visualization of what scene they want in a movie. Or when you’re thinking about a large scale, like a public art, large scale kind of installation, you could start having DALL-E generate things and go, “Oh, this version of it.”
And again, doesn’t mean you’re going to do just the thing that DALL-E generates. But it becomes a fast loop by suddenly you had 200 prototypes where you said, well out of 352 and 78, those had the elements that I’m going to bring together in the thing that’s even more amazing than the thing I could have done before. And I think this is true across all of these things.
And so that gets to the consumer part, or the everyday individual part, I do think that all of these tools can be more used by everyday individuals. Doesn’t mean everyone’s going to do it. Doesn’t mean everyone has to do it right away. I think it’ll become a professional standard. If you do it professionally, it’ll be like, well if I’m trying to do graphic design and I don’t use Figma, or I don’t use Photoshop, then it’d be like, “Eh, I need to have those tools as a baseline.” But everyday individuals also can create something like a Valentine’s Card or something else as well.
SM:
Yeah. I would add to that. So as Reid was going, I was thinking of what he was describing, which I’d put in the bucket of new products and what will feel like new products and new experiences to consumers. And I think there’s a lot that’s going to happen there.
The other kind of framing that Reid and I often talk about is, like I was thinking about the Jetsons analogy. And one thing that we’ve talked a lot about in Silicon Valley is this idea of natural language interface to computing. In many ways, the way we use computers is super clunky. And I think because of some of these large language models, you will actually be able to just talk to your computer.
Now you may type, you may speak out loud, but you’ll speak in natural language. We’re seeing the early signs of that with how you generate images on DALL-E, or generate text with GPT-3. But you’ll have tools that will be able to do things like, “Hey, my mom’s birthday is next Wednesday. Can you please organize a gift?”
And the model with the combination of actuation will come back and say, “Hey, Chris, here are three things we know your mom likes. Pick the one you want. We’ll use this API to actually order it for you, write a handwritten card, and send it to her.” And so in that way, we’ll have this new interface to computing that will be quite interesting. And I think people will adopt that. That’s kind of one bucket.
But then the second thing is that AI’s already pervasive in a lot of the technologies we use and we just don’t realize (or many of us may not realize it). So at Greylock, we’re investors in ByteDance. And many of us have used TikTok and there are many things that make TikTok a great consumer experience, but one of them is just how good the relevance is. I certainly find myself scrolling it for way too long because of how relevant the content it’s showing to me is. And that’s because of a lot of investment in AI to actually drive that real time relevance and model updating. And we see that in the context of ByteDance. We see that in the context of something like DoorDash that suggests to you a new pizza restaurant, because it turns out you really like Neapolitan pizza.
And so I think it’s like we will see these new products, but I also think lots of consumers are and will in an increased way, be interacting with these AI advances without fully realizing it.
CY:
And that’s the thing. All these products, all these services, they’re so much better than we imagined them in advance. And something like TikTok has this magical ability to draw my daughter in and just watch it endlessly.
And you say, oh yeah, there’s some silly videos. Well, why would anyone watch that for an hour? And yet when you experience it, it’s like, oh my gosh, there’s no way to avoid it. The AI is so powerful. To which of course my response is I, for one, welcome our new robot overlords.
RH:
Yes. That’s the classic tongue in cheek, Silicon Valley line. And I do think that one of the things that Saam’s saying here is particularly important, which is that there will be some amazing products that you’ll be able to interface with and that’s that generation of magic. But it’s also going to re conceptualize a whole wide variety of services. And there’s already a bunch of services that are doing it.
I mean, to some degree, search is powered by a bunch of different kinds of AI techniques for relevance and how that works. The news feeds and various things including TikTok, and others, use this. As an example, there’s already been for years an ability to upload a photo and then make the photo like an Impressionist or make the photo Cubist, or do other kinds of things as ways of doing that. And that already exists within all these things. And they’re all part of this new paradigm of AI tools.
And so whatever thing, not only are there going to be new things, but everything you currently do is ultimately going to be powered and accelerated. Now, it even gets down to the connection of atoms and bits. Because obviously the software transformation of the world, the using of a bunch of data, and using AI as kind of the exponential amplifier and all this stuff.
Well that even begins to play to atoms in how you are doing it. Like, for example, one of the things you already have happening is a bunch of different AI efforts led primarily by DeepMind, they’re doing protein folding. That will lead to all kinds of things that are possible. Evolution or mRNA vaccines, or other kinds of precision medicine. That’s one form of bits to atoms. The kind of part of what’s already happening with major pieces of machinery, including planes and so forth, is you have a whole bunch of monitors on all parts of it. And it will actually do, will monitor, is it safe? Does it need preventative maintenance? Et cetera, et cetera. How is that working?
You’ve already had, actually, designs of like airfoils that come through a kind of simulation and use of these AI techniques. Rather than purely just the physical thing of a tube with a fan blowing through it, you use an iteration around simulation and kind of the laws of physics in order to hone new, interesting designs on it.
And so the very infrastructure, the fabric of a bunch of things that we’re already doing, will be reinvented, renovated, revolutionized, exactly as Saam’s talking about through a variety of AI techniques. All the way into the world of atoms from bits. And obviously bits is where you start and bits is where we tend to do, to where we anchor our work at Greylock. But it’ll have a broad reaching set of transformations and improvements across society.
“The very infrastructure – the fabric of a bunch of things that we’re already doing – will be reinvented, renovated, and revolutionized.”
CY:
Absolutely. Now thinking about investing, which is something that obviously is on your minds as partners at Greylock, you’ve partnered with a lot of these AI companies. But for every one that you invest in there’s dozens or 100s more that are out there looking to raise money. How do you decide where to focus? And where are you seeing the most promising activity?
RH:
Well, both Saam and I have made a number of investments. And the two that I’ve worked on, one directly, one with Saam, Inflection and Adept, both aren’t super clear yet with the entire market as to what they’re doing. Because they have some great ideas and the Inflection point it’s Mustafa Suleyman, former of co-founder of DeepMind. At Adept, it’s David Luan, from OpenAI and Google and a bunch of other great people from Google kind of doing this. And then some others.
And they both have a really good solid conception of building a unique product relative to the other things that we saw in the field. And it was one of the things that becomes kind of a lens for looking at this. Because there’s some stuff with just a standard like, okay, do you have a good go to market? Do you have a really talented team? Do you have a sense of what competitive differentiation looks like? Do you have a well conceived business that might have a competitive moat? Or an ability to, once you establish, a position that’s really good? On the other hand, you also have to understand this technology. It’s a rare talent set. What’s really possible with it? What are the things that are going to create some differentiation?
SM:
Yeah. I think to piggyback off of Reid, when we think about AI and the enterprise, there’s roughly two buckets, applications and infrastructure. And maybe I can comment on each. So on the application side, it’s early days, we think a whole new wave of SaaS and business applications is going to be built as AI native or applied AI companies. And the real idea here is take any function and can you leverage AI on top of data sets to really drive strong business to ROI? And so to give you examples, on the cyber side, we think it’s extremely fertile. Because in cyber you have so much telemetry and so much noise to sift through. And like the discriminative abilities of AI are actually really useful in kind of parsing the signal there.
And so one example would be Abnormal AI, a company we’re investors in at Greylock, which is building a next generation email security platform. And they’re training on email data and organizational data. And they build models of, okay, Reid – how does Reid typically behave on email? Versus how does Saam typically behave? Everything from language topics. And then when there’s anomalies, they identify that for security teams. And that’s how they solve different types of attacks.
In the sales contacts, companies like Cresta, leveraging generative techniques. So at Cresta kind of the mission is how do we make every salesperson 100 times more effective? And so imagine software that’s kind of your co-pilot as you’re a sales rep at AT&T talking to a customer. Can we help generate suggestions for what you can say to the customer to help drive the business outcome you’re trying to drive? Whether that’s a new sale or preventing a customer from churning.
So I think there’s a lot to do in applications. We’re looking for applications where there’s a strong connection between the model’s output and business ROI. Because then it makes it very easily to contextualize the power of AI to the customer. And then I’d add two additional things we look for and they both relate to data.
So one is data readiness and availability. So you have to train these models, so we want to back products where they’re entering domains or verticals where there is a lot of data; that the customer has in a format that the product can actually train on. The second piece of data is that human feedback’s very important, so [we’re looking for] founders who can really craft these AI products that humans actually want to use and interact with. Because that interaction ends up driving back into the model and making the model more performative over time. So that’s the application side of things.
On the infrastructure side, it’s all about enabling large enterprises to take better advantage of AI. And here, I kind of think of three main thrusts: One is if you think about that pipeline that we talked about earlier, Chris, from data to model and production, doing inference and monitoring for things like bias and explain-ability, each part of that pipeline is an opportunity. And so today we have investments in companies like Snorkel that focus on data management and an automated approach to labeling data, to make it ready for machine learning. Or companies like TruEra who are on the other side of the pipeline. Where when you have your model running, making loan decisions, and you need to be able to explain and audit those decisions, TruEra enables companies to do that. So that’s kind of one bucket of infrastructure.
The second is actually empowering and making builders – data scientists and machine learning engineers – more productive. So you can think of collaboration tools for these people, experiment tracking, all sorts of things as this becomes a much more important user demographic inside large companies.
And then the third is democratizing AI. And what we mean by that is I think about the analogy of if you take Tableau. And lots of people inside companies know how to use Tableau to get a sense of what’s happened in the past in their data. And what’s the equivalent for what’s going to happen in the future? And so if I have a business analyst who may not know what a hyper parameter is, but has a predictive problem, can we build tooling to actually enable those people to harness the power of AI? That’s a big thrust for us as well.
CY:
Now, speaking of the enterprise, it feels like there’s a couple of different kinds of companies. There’s these giants like Google and Microsoft, they have big in-house AI efforts. They’re trying to play in this space. But then other enterprises are looking to buy rather than build. What’s the difference in how AI fits into the tech stacks of these different kinds of companies?
RH:
One of the things that actually I think is pretty interesting to look at as an example, is what Microsoft and OpenAI are doing together.
So on one part of it, Microsoft is trying to build a platform in Azure using the kinds of products that OpenAI is doing to make sure that it has the best compute fabric, the infrastructure for kind of supercomputing and ways of doing it. And then kind of is enabling a set of what they call cognitive services in order to enable a bunch of AI generated applications within Azure.
And on the other hand, they’re also releasing some first party products, both with Microsoft and also having OpenAI do it as an exemplar. And there’s kind of like three key things that I kind of point out. One is, which we’ve referred to somewhat already, which is GBD, which has currently GPT-3, which is what’s out from last year. But there will be a GPT-4 that is coming. And it has not only various forms of language generation, but also gives you translation and a bunch of other stuff basically for free. It’s one of the things that’s kind of part of the magic of these.
One of the parts of it is you say, well, you train it on the internet and text. But then you fine tune it on code. And you have the co-pilot product, which last year’s model with 100,000 developers had developers accepting 35% of the coding suggestions. And these aren’t like finish the variable name. It’s like you’re trying to do this kind of activity that you want to import into this library, use this library, write this code. Whether it’s a sort, a data processing, any kind of algorithm, any kinds of things and doing that sort of thing.
And then DALL-E, which we’ve already mentioned, which is doing image generation. And part of the reason why I went out and did these essays on how important DALL-E will be, is I actually think that there’s going to be a landmark here of the world of graphic design that’s pre DALL-E and post DALL-E. Just kind of like pre Adobe Photoshop, post Adobe Photoshop. Pre Figma, post Figma, as a way of doing this. And I think that’s all part of the kinds of things that will be coming out as also first party applications in addition to third party fabric.
SM:
Maybe to add to that lens from the enterprise perspective, we think about this bifurcation, Chris, as you alluded to around build versus buy. And our mental model for it is that build is when a company’s really building something that’s their core competency. So you could take the most extreme view and say Google search. Like Google search every additional point of accuracy and effectiveness is critical in the core competency of that business. And so that’s not really an opportunity for third party vendors to come in and sell tooling against it. And that’s a very extreme example. But you take banks, for example, banks who are underwriting credit products. These are areas where these companies are investing in their own internal data science teams. They want to eke out every additional point of performance.There’s some opportunities at the edges of that to sell highly specialized infrastructure. But we’ve stayed away from that because it’s a more niche opportunity.
On the buy side, though, the lens we use is where are their horizontal business workflows that are important? But not the core competency of any one organization. So document processing and extraction, contact center AI. There are these core primitives that are shared by how these enterprises operate and are important in great application areas for AI, but not core to any single customer. And that’s where there’s really an opportunity for these third party applications to come in and sell and companies will buy. And that’s where we look to invest.
CY:
And it sounds like when you talk about infrastructure, it’s almost like a classic platform business model. Now it’s all of a sudden you’re enabling so much more and all the people who build on top of your primitives are increasing their value.
SM:
That’s right. That’s exactly right.
RH:
I mean, part of one of the reasons why AI is super interesting, and one of the things that Saam and I, and others at Greylock focus on – of course, why we also are paying attention to Web3 – is, new technologies become new platforms for either the reinvention of existing applications or the generation of new applications. And part of the reason why we’ve been kind of going through not just the discriminatory models, but the generative models on this, is the number of kind of new kinds of applications that just simply weren’t there before now. And everyone naturally tends to go consumer in their thinking, because that’s the more broad. But even the enterprise is magical. And is amazingly interesting.
CY:
Now speaking of that consumer side, I’m a creator. I have a degree in design from Stanford. So I did a bunch of studio art. I have a degree in creative writing and obviously I’m still an author. And so I’m really excited about things like GPT-3 and DALL-E. So these are just astonishing. They’ve left behind these crude and primitive, “Hey, this is a cat or a chat bot for some sort of customer service.” How did we get here? How did we get to the point where we’re actually creating real content with AI?
“New technologies become new platforms for either the reinvention of existing applications or the generation of new applications.”
RH:
Well, part of the thing that happened is – and by the way, most experts didn’t actually predict that the large language models and foundational models would get as magical as they would – actually most of the pieces of technology have existed for several decades.
But what happened is when you threw enough compute at it, and you threw enough data at it, and you added in some other mechanisms. For example, how to really use all that data, whether it’s the transformer model, or the self play model. That all of a sudden on just intensive amounts of compute over the data, you end up with kind of magical applications.
And so for example, one of the things that happens with GPT-3 is you can feed it things like prize essay questions from the All Souls Prize Fellowship in Oxford University. And it says there is no Marx without Lenin, discuss. Or Schrodinger’s cat challenges our concept of reality, true/false. And it will generate something that’s actually, in fact, human and coherent and interesting. That doesn’t mean that it’s always an A-plus essay. Matter of fact, actually sometimes it’s kind of a B-plus essay, but even B plus is amazing. It wasn’t doable before. And it comes from the fact that it’s like this huge model that’s been generated, that’s been trained on generativity relative to what’s interesting. And you say, well, there’s at least anecdotal parallels to what human beings do. Because you kind of say, how do you teach writing? And so it’s like, “Well, okay, oh, See Spot Run.” And then it’s like, you start going, “Okay, what’s interesting?” And people say, “Oh, this is a great use of language. This is a great way. You’ve now spoken. You’ve now written the right way. And then we generate.”
Now, whether or not it’s anywhere close to the same mechanisms that we use to be generative, there’s some dispute on. I tend to think it’s inspired by the way we do it. But it’s actually, in fact, very different. Among them, the more geeky thing, is part of what these large language models do is they deploy huge amounts of ability of scale and electricity in order to do it.
On a kind of cognitive capability as you were (like an IQ point per watt), our brains still function a lot better per watt. Per unit of electricity, we’re much more generative and so forth. It’s just that this other architecture allows you to apply scale in a really interesting way, which allows you to generate things that you previously couldn’t generate.
CY:
And that’s one of those fascinating things. Efficiency is great, but scale has its own power as we’ve discussed in the past.
RH:
Yep. And a nice little bow, Chris, to our work in Blitzscaling and everything else, which I saw you going there.
CY:
I was teeing it up for you.
RH:
Yes.
CY:
Now, obviously we’re getting pretty excited about this. That’s because to be in this industry, you kind of have to be an optimist, a techno optimist, if you will. But there’s also a dark side to these things. There’s a lot of ethical considerations to take into account when developing AI. So what are some of these top ethical concerns in the field today?
SM:
That’s a really important area. So on the enterprise side, there’s like two buckets of challenges. One is how do you know that the data you’re training your model on is actually representative of the real world? And also preserves the underlying privacy of whatever you’re training on?
And so I think that’s a really important area and we see different tools and projects that have emerged that enable you to understand distributions of data sets, enable you to do things like create synthetic forms of your data set. So that actually a data scientist can go in and play with the data and look at it, but not actually compromise any of the underlying privacy of the data. And I think that’s actually really important to take advantage of these data sets that live in the enterprise and have such strong predictive power.
Then on the actual inference side, the key thing is actually being able to explain why a model’s making a particular prediction. And then once you have that fundamental explanation, you want to apply different lenses to it. So for example, the canonical example I think about is the loan approved or disapproved example. So you have a model, you feed it some data about a borrower and the model comes back and says, “This loan’s been denied.”
Now, there are a list of important regulations that actually dictate the kinds of things that you can deny a loan for that are kosher versus not. And then the question is, did the model adhere to that? And so in order to answer that, you need to actually be able to explain why the model denied something. What were the different variables and parameters around the data vector, if you will, of the applicant that led to that decision? And is that fair? Or is that ethical? Might be another way to approach that.
I actually think a lot of the real world deployment of AI in areas where it could be very useful, is rightfully blocked because we don’t yet, as an industry, have what the answer here fully is. And what we have to get to is an agreed upon definition of explain-ability. Agreed upon standards on how we actually audit these decisions. And then a way to apply an agreed upon set of standards from a fairness and bias perspective on top of these explanations. And there’s lots of good work happening, but it’s still early days there.
“A lot of the real world deployment of AI in areas where it could be very useful, is rightfully blocked because we don’t yet, as an industry, have what the answer here fully is.”
RH:
Yeah. Plus one on everything Saam said. And then expanding some: I mean, one part of this is that as AI, currently AI is very difficult to actually look under the hood and know what’s going on. Unlike when you write an if/then kind of code. If a person is 40 years or older and has a FICO score of X, then blah, blah, blah. Then you go, okay, I know how it’s operating.
These large models are intensely pattern coordinators, but they’re very hard to see exactly what they’re doing. And so you say, “Well, is it being fair? Is it being just? Is it operating? When it’s in unusual circumstances, how is it operating?” And this gets to another area that we’ve been investing in at Greylock, which is autonomous vehicles. Things like Aurora, things like Nuro, things like Nauto. There’s also Uber for trucks like Convoy. But you look at these areas and you say, “Okay, well, what are the AI and safety implications here?”
It’s like, well, you really want to make sure that it’s great relative to human life, and relative to creating traffic jams on highways and trucks and so forth. Now, one of the unfortunate things is that human beings tend to want to do this as if it were perfect safety. And you’re like, well, actually in fact, about 40,000 people a year die in the US in car accidents today – and by the way, part of the data from Nauto shows that a huge portion of that is the 10% of worst drivers. So your standard of, if you could actually get to like everyone to just the middle point and the autonomous vehicles got everyone to the middle point, you’d save 10s of 1000s of lives, everything. Even if you still had 3,000 deaths or 5,000 deaths per thing.
So you have to balance out what that safety is. It’s really important to have safety in human lives. But like, if you said, well, this one is eight times safer, and is going to save seven lives, for everyone who does that, would be something that we should want.
Now, of course, part of what gets funny about this is that people say, you ask people, you say, “Well, what would you like people doing?” It’s like, “Well, I’d like everyone else to be in an autonomous vehicle car that emphasizes the maximum safety of life. And I’d like the one I’m in to be safe in my life.” And you’re like, okay, so you have to work out these kinds of social issues.
And then obviously there’s kind of the unintended consequences. And this is part of the reason why most Hollywood films tend to go, “The robots are coming for you.” And in fact I actually think frequently that the question is, “Can the robots get here soon enough?”
Can we get here soon enough to help us with our elderly care problem? Can they help us with our new amplifiers of human manufacturing? Can they help us with kind of dangerous kind of cleanup areas? Whether it’s wildfires or other kinds of things in terms of how to have things happen.
But you still have to look at whether there unintended cyber kind of consequences? So if you said, “Well, we have a really good cyber defense thing.” But what if the cyber defense thing breaks? Or somehow breaks the network? And so that would be an important issue. And so you have to look at what frequently is referred to as the paperclip problem, which is it’s maximizing the wrong function and that has very bad side consequences.
You have to look at all of this stuff. Now, one of the things that I think that people don’t realize, is that it’s, again, just like the autonomous vehicles, which most people who are doing this stuff are thinking about safety stuff, are trying to do it. Doesn’t mean it’s perfect.
And what we’re trying to do is figure out, well, what’s the best safety we can get while still getting all the improvement? And so, like I wrote an essay, oh gosh, might even have been almost 10 years ago now. That was saying not only we should want the autonomous vehicles to be here as soon as possible. Because today’s autonomous vehicle technology from Neuro and Aurora and others, would actually in fact, already be saving tons of lives if it was deployed. Obviously there’s a bunch of work to still do.
But anyway, those are a whole bunch of ways of thinking about the ethical considerations. And the fact that people are actually in fact, engaging it, thinking about it, talking about it, trying to work on it. Still a bunch of work to be done.
“Obviously there’s the unintended consequences. And this is part of the reason why most Hollywood films tend to go, ‘The robots are coming for you.’ And, in fact, I actually think, frequently, that the question is, ‘Can the robots get here soon enough?'”
SM:
I’d add, I’d be remiss, in addition to AI, I spent a lot of time on security at Greylock. And I’d be remiss not to add on the security angle here. So I think there are a number of potential threat factors and opportunities for adversarial attacks on machine learning systems. And that’s an area that we’re paying a lot of attention to, and we think there’s opportunity to strengthen.
And two of the things that, for example, we think about. One is on the data poisoning side. So if you think about these models, they’re entirely informed by the data on which they’re trained. And so if an attacker comes in and actually poisons those data sets, you can create a model that’s now making predictions that are anchored in a really adverse way. And so that’s one big problem and an area where a lot of work is going on. And we’ve looked at projects.
Another area is on the what’s called model extraction side, which is you have a black box machine learning system that was trained on some data. And let’s say there’s sensitivity around that data, or there’s privacy issues around that data. And now an attacker comes in and keeps hitting the model for different inferences, with different inference requests, with the goal of backing out some of the underlying semantics of the data, the model was trained on.
And so I just described two attack factors. There are many, but as these systems become more ubiquitous, the surface area of risk also increases. And so it’s like an area we as an industry have to spend a lot of time thinking about. And we at Greylock think about, and meet with entrepreneurs who are trying to kind of solve these problems as well.
CY:
As I hear this, taking heart from it, because it sounds like people are really thinking about it very carefully. But I also can’t help, but picture the next Terminator movie with octogenarian Arnold Schwarzenegger, where he’s working in an elder care home. And he’s telling them, “Come with me, if you want to live a longer and healthier life.” That’s what I’m picturing now.
So one final question then, which is to take a look into the future. As AI continues to evolve, what innovations do you two hope to see in the near and far future?
RH:
Well, one of the things I think is really fun about the whole venture business is while we kind of work on our prepared DCs, we talk with great entrepreneurs. We talk amongst ourselves, we have a bunch of things. Obviously, we are being surprised by things that we don’t see that are really amazing. This is a little bit like the sooner and stranger.
And that’s one of the delights of the job, is finding those entrepreneurs where she or he might be like, I’ve got this idea and vision. You’re like, wow, I never really thought about that. It was kind of like when Brian, Joe, and Nate were talking to me about Airbnb, my first investment for Greylock. And I think that’s the kind of thing that, as we hope to see, we hope to see that amazing entrepreneur coming with something.
And the reason why I open that, because that’s the most important thing I think you do as an investor, is have a prepared and open mind for finding the transformative idea, the new idea, that’s out there in the world. And those are the kinds of technologists and inventors and entrepreneurs.
But I do think that what you’re going to see is the kind of the set of things, where you begin to say, “Well, what are the really major areas that matter to human beings?” And it’s like, well, medicine matters. I think you’ll see a bunch of stuff that’ll help with precision medicine and other kinds of things and that.
I think education will be similar. I think there’ll be a bunch of things that kind of lead to like, okay, how do you optimize energy in the grid? I think there will even be impacts on kind of climate change, relative to maybe amplifications of how you model a fusion environment, or fission environment, and make that a lot better.
These are just some of the things that I think you will begin to possibly see. And none of that even begins to approach the infrastructure of which our society works, which is part of what makes actually, in fact, enterprise investing so interesting.
SM:
Yeah. And I’d second what Reid says, which is I think the privilege of what we get to do is we get to meet entrepreneurs who are building that future and we dream alongside them. So we look to them to navigate us. That said, there’s a lot we’re excited about.
I mean, on the enterprise side, there’s a lot of narrow applications that I think are really important. So Reid mentioned medicine. On the software side, you think about the electronic health record systems that these hospitals operate on. And when you go in and see your physician and they’re writing down notes on the encounter, and then they’re kicking off a bunch of workflows based on what you need coming out of that encounter. All of that, we’re investors in a company called Notable Health. That’s kind of automating that end to end workflow.
There’s a lot of these kinds of “Pick your vertical, pick your function.” And AI should make the process much better. And I think that’s going to pervade everything over the coming years. But the other thing I noticed, automation is an area we, as an industry, we’ve just talked about a lot. And there’s these robotic process automation companies that have come out and different forms of kind of automating these discrete workflows. But to date, it’s all been brittle and narrow and very kind of workflow specific.
I think the combination of large language models – some of the advancements in actuation and program synthesis and the ability to operate across modalities of data – I feel like we have the now core technical building blocks where we can really deliver on this vision of “How do we make the knowledge worker 100 times as proactive?
And how do all three of us kind of show up at our desk and have something on our computer that’s observing what we’re doing. Saying, “Hey, Reid, I’m noticing you’re doing this thing over and over again. Can I help you do it?” But then it starts doing it and makes a mistake with natural language. Reid can correct it. It’s now learned, so the next time it doesn’t make a mistake. And now Reid’s 10 times as effective because he has this true AI assistant or co-pilot. I think that is something we’re going to see happen on the bit side, and on the enterprise side.
We’re investors in a company which is using models for prediction of RNA structure, to design a whole new class of RNA targeting therapeutics. That could be transformative to a bunch of different areas of pharma.
Reid mentioned Aurora and Nuro. The advancements there and you know how that’s going to transform, not just self-driving, but the derivative impacts of that across the entire supply chain. I think it’s going to be very, very impactful, and I’m really excited to see what the next generation of entrepreneurs and great companies come up with.
CY:
Well, that brings us to the end of today’s discussion. A lot of fascinating topics. We just scratched the surface. And I’m going to be very excited to hear you guys dig deeper into all of them with your guests in the coming weeks. Reid, Saam, thank you so much for joining us today.
RH:
Chris, thank you as always.
SM:
Thanks, Chris.
CY:
And for our listeners out there, you can subscribe to Greymatter wherever you get your podcasts. And you can find all our content on our website, Greylock.com. You can also follow us on Twitter, at GreylockVC. I’m Chris Yeh, and thank you for listening.